Research Article | OPEN ACCESS
Prediction of Pork Fatty Acid Content using Image Texture Features
1, 2Xin Sun, 1David Newman, 1Jennifer Young, 2Yu Zhang and 1Eric Berg
1Department of Animal Sciences, North Dakota State University, Fargo, ND 58102, USA
2Department of Engineering, Nanjing Agricultural University, Nanjing 210031, China
Advance Journal of Food Science and Technology 2016 11:644-647
Received: May 13, 2016 | Accepted: June 28, 2016 | Published: December 15, 2016
Abstract
The objective of this study was to investigate the usefulness of image texture features obtained from fresh (never frozen) pork backfat for the prediction of fatty acid content and Iodine Value (IV). Five image texture features (directionality, contrast, roughness, heterogeneity and line-likeness) were extracted from cross-sectional images of 9pork loin chops with overlying subcutaneous fat. Texture features were extracted from images obtained on the subcutaneous fat using a digital camera. A full fatty acid profile was determined for each subcutaneous fat sample using AOAC and AOCS official methods. Linear and stepwise regression methods were utilized to establish the prediction models for oleic, linoleic and linolenic fatty acids and IV. Linear regression analyses produced higher coefficients of determination (R2) for all 3fatty acids. Linear regression models for linoleic and linolenic acid generated an R2 of 0.95. These preliminary findings suggest potential for use of image texture features for prediction of pork fattyacid values and subsequent pork fat quality.
Keywords:
Fatty acid, image processing, iodine value, prediction, texture feature,
References
-
AOCS, 1998. Method Cd 3b-76: Saponification Value. In: Firestone, D. (Ed.), Official Methods and Recommended Practices of the American Oil Chemists' Society, 5th Edn., American Oil Chemists' Society, Champaign, Illinois, USA.
-
Chandraratne, M.R., D. Kulasiri, C. Frampton, S. Samarasinghe and R. Bickerstaffe, 2006a. Prediction of lamb carcass grades using features extracted from lamb chop images. J. Food Eng., 74(1): 116-124.
CrossRef Direct Link -
Chandraratne, M.R., S. Samarasinghe, D. Kulasiri and R. Bickerstaffe, 2006b. Prediction of lamb tenderness using image surface texture features. J. Food Eng., 77(3): 492-499.
CrossRef Direct Link -
Chandraratne, M.R., D. Kulasiri and S. Samarasinghe, 2007. Classification of lamb carcass using machine vision: Comparison of statistical and neural network analyses. J. Food Eng., 82(1): 26-34.
CrossRef Direct Link -
Chen, K., X. Sun and C. Qin, 2010. Color grading of beef fat by using computer vision and support vector machine. Comput. Electron. Agr., 70(1): 27-32.
CrossRef -
Chmiel, M., M. Slowinski and K. Dasiewicz, 2011a. Lightness of the color measured by computer image analysis as a factor for assessing the quality of pork meat. Meat Sci., 88(3): 566-570.
CrossRef PMid:21392895 -
Chmiel, M., M. Slowinski and K. Dasiewicz, 2011b. Application of computer vision systems for estimation of fat content in poultry meat. Food Control, 22(8): 1424-1427.
CrossRef Direct Link -
De Marchi, M., R. Riovanto, M. Penasa and M. Cassandro, 2012. At-line prediction of fatty acid profile in chicken breast using near infrared reflectance spectroscopy. Meat Sci., 90(3): 653-657.
CrossRef PMid:22082651 Direct Link -
Girolami, A., F. Napolitano, D. Faraone and A. Braghieri, 2013. Measurement of meat color using a computer vision system. Meat Sci., 93(1): 111-118.
CrossRef PMid:22981646 Direct Link -
González-Martín, I., C. González-Pérez, N. Alvarez-García and J.M. González-Cabrera, 2005. On-line determination of fatty acid composition in intramuscular fat of Iberian pork loin by NIRs with a remote reflectance fibre optic probe. Meat Sci., 69(2): 243-248. http://www.sciencedirect.com/science/article/pii/S0309174004001810.
CrossRef PMid:22062814 Direct Link -
Hallenstvedt, E., M. Øverland, A. Rehnberg, N.P. Kjos and M. Thomassen, 2012. Sensory quality of short- and long-term frozen stored pork products. Influence of diets varying in polyunsaturated fatty acid (PUFA) content and iodine value. Meat Sci., 90(1): 244-251. http://www.ncbi.nlm.nih.gov/pubmed/21820249.
CrossRef PMid:21820249 Direct Link -
Ripoche, A. and A.S. Guillard, 2001. Determination of fatty acid composition of pork fat by Fourier transform infrared spectroscopy. Meat Sci., 58(3): 299-304.
CrossRef Direct Link -
Sun, X., K.J. Chen, K.R. Maddock-Carlin, V.L. Anderson, A.N. Lepper et al., 2012. Predicting beef tenderness using color and multispectral image texture features. Meat Sci., 92(4): 386-393.
CrossRef PMid:22647652 -
Sun, X., K.J. Chen, E.P. Berg, D.J. Newman, C.A. Schwartz et al., 2014. Prediction of troponin-T degradation using color image texture features in 10d aged beef longissimus steaks. Meat Sci., 96(2 Pt A): 837-842.
CrossRef PMid:24200578 -
Tamura, H., S. Mori and T. Yamawaki, 1978. Textural features corresponding to visual perception. IEEE T. Syst. Man Cyb., 8(6): 460-473.
CrossRef
Competing interests
The authors have no competing interests.
Open Access Policy
This article is distributed under the terms of the Creative Commons Attribution 4.0 International License (http://creativecommons.org/licenses/by/4.0/), which permits unrestricted use, distribution, and reproduction in any medium, provided you give appropriate credit to the original author(s) and the source, provide a link to the Creative Commons license, and indicate if changes were made.
Copyright
The authors have no competing interests.
|
|
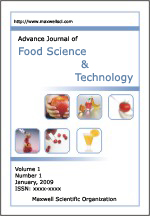 |
ISSN (Online): 2042-4876
ISSN (Print): 2042-4868 |
 |
Information |
|
|
|
Sales & Services |
|
|
|