Research Article | OPEN ACCESS
Prediction Model of Thermal Properties of Fruits and Vegetables Based on Random Forest and Fusion Model
Xi Xie and Weizhong Jiang
School of Electrical and Information, Jinan University, Zhuhai, 519070, China
Advance Journal of Food Science and Technology 2016 11:609-616
Received: March ‎10, ‎2016 | Accepted: May ‎23, ‎2016 | Published: December 15, 2016
Abstract
This study is aimed to model the complicated correlation between physicochemical property and thermal property of fruits and vegetables. And this study predicts thermal property values with higher accuracy based on fusion model and Pearson correlation analysis. Thermal property is important for the storage and transportation of fruits and vegetables. And it’s an important factor of fruits and vegetables fresh-keeping. To design a fresh-keeping device, it’s necessary to measure the thermal properties of fruits and vegetables. Some people use complicated devices to measure thermal properties directly or establish physical thermal model to analyze thermal properties. But it’s difficult to use only physical thermal model to express the complicated correlation between physicochemical properties and thermal properties. Leaning machine is a new way to model the complicated correlation among multiple dimensions attributes. At first, this study uses Pearson correlation analysis to analyze the correlation between physicochemical property and thermal property. This step is to choose predictors which will be used to predict the thermal properties. This study uses BP neural network, random forest and GBDT algorithm to predict thermal properties of fruits and vegetables. And after testing models with 700 sets of original data, the test result shows that all of these three models have good performance. To get higher accuracy of prediction, this study uses BP NN and random forest to establish fusion model which means it uses random forest to fuse the prediction result of random forest, BP neural network and the original predictors. The performance of fusion model is 89.3% (R2) for prediction of Thermal conductivity and 96.3% (R-square) for prediction of Freezing point. The test result shows that fusion model has better performance and higher accuracy to predict thermal properties of fruits and vegetables. This study was conducted in Electrical and Information School, Jinan University from Apr. 15th 2015 to Mar. 1st 2016.
Keywords:
Fruits and vegetables, fusion model, physicochemical properties, Pearson correlation analysis, random forest, thermal properties,
References
-
Breiman, L., 2001. Random forests. Mach. Learn., 45(1): 5-32.
CrossRef Direct Link -
Brillante, L., F. Gaiotti, L. Lovat, S. Vincenzi, S. Giacosa, F. Torchio, S.R. Segade, L. Rolle and D. Tomasi, 2015. Investigating the use of gradient boosting machine, random forest and their ensemble to predict skin flavonoid content from berry physical–mechanical characteristics in wine grapes. Comput. Electron. Agr., 117: 186-193.
-
Cao, Z.F., 2014. Study on optimization of random forests algorithm. Ph.D. Thesis, Capital University of Economics and Business, Henan.
-
Cheng, Y.J., B. Liu, Q. Wang, J. Liu and R.X. Chai, 2006. Investigation and comparation of fruits and vegetables thermophysical property. Storage Process, 6(3): 26-28.
-
Jian, Y.J., 2013. Research on correlation rules mining algorithm based on matrix. M.S. Thesis, Lanzhou University, Gansu.
-
Maloney, K.O., M. Schmid and D.E. Weller, 2012. Applying additive modelling and gradient boosting to assess the effects of watershed and reach characteristics on riverine assemblages. Method. Ecol. Evol., 3(1): 116-128.
CrossRef Direct Link -
Sablani, S.S. and M. Shafiur Rahman, 2003. Using neural networks to predict thermal conductivity of food as a function of moisture content, temperature and apparent porosity. Food Res. Int., 36(6): 617-623.
CrossRef Direct Link -
Strobl, C., A.L. Boulesteix, A. Zeileis and T. Hothorn, 2007. Bias in random forest variable importance measures: Illustrations, sources and a solution. BMC Bioinformatics, 8(1): 1-25.
CrossRef PMid:17254353 PMCid:PMC1796903 Direct Link -
Zhang, M., 2005. Study of thermal conductivity measurement system and temperature field simulation of postharvest fruits and vegetables. Ph.D. Thesis, Henan Agricultural University, Henan.
Direct Link -
Zhang, M., Z.Y. Zhong, L. Yang, H.Z. Zhao, J.H. Chen and Z.H. Che, 2010. Prediction model of thermal conductivities of fruits and vegetables based on BP neural networks. T. CSAE, 41: 117-121.
-
Zhong, Z.Y., 2010. Relationships between thermo-physical properties and physiological and biochemical parameters of fruits and vegetables and neural network prediction model on thermo-physical properties. M.Sc. Thesis, Shanghai Ocean University, Shanghai.
Direct Link
Competing interests
The authors have no competing interests.
Open Access Policy
This article is distributed under the terms of the Creative Commons Attribution 4.0 International License (http://creativecommons.org/licenses/by/4.0/), which permits unrestricted use, distribution, and reproduction in any medium, provided you give appropriate credit to the original author(s) and the source, provide a link to the Creative Commons license, and indicate if changes were made.
Copyright
The authors have no competing interests.
|
|
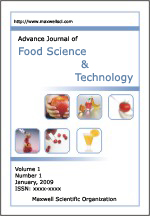 |
ISSN (Online): 2042-4876
ISSN (Print): 2042-4868 |
 |
Information |
|
|
|
Sales & Services |
|
|
|