Research Article | OPEN ACCESS
Prediction of Microwave-Assisted Extraction to Determine Zinc in Fish Muscles Using Support Vector Machine and Artificial Neural Network
1ZhihanXu, 2JinkunYao and 3YimengWang
1College of Light Industry, Textile and Food Engineering
2College of Chemistry
3College of Manufacturing Science and Engineering, Sichuan University, Chengdu, China
Advance Journal of Food Science and Technology 2016 9:680-686
Received: May 25, 2015 | Accepted: June 19, 2015 | Published: March 25, 2016
Abstract
In this study, the support vector machine and the artificial neural network are adopted in the microwave-assisted extraction method to determine the amount of zinc in fish muscle samples. In the experiment, the irradiation power, irradiation time, nitric acid concentration and temperature are set as independent variables while the amount of zinc was considered as a function of the four factors. By comparing the RMS error and the training time of the support vector machine and the artificial neural network, the most suitable predicting model can be determined. The results show that the MLFN model with 7 nodes performed the best with the lowest RMS error of 1.21 and 100% prediction accuracy.
Keywords:
Artificial neural networks, microwave-assisted extraction, predicting accuracy, support vector machine,
References
-
Bas, D. and I.H. Boyaci, 2007. Modeling and optimization II: Comparison of estimation capabilities of response surface methodology with artificial neural networks in a biochemical reaction. J. Food Eng., 78(3): 846-854.
CrossRef -
Basri, M., R.R. Zaliha, A. Ebrahimpour, A.B. Salleh, E.R. Gunawan and M.B. Abdul-Rahman, 2007. Comparison of estimation capabilities of Response Surface Methodology (RSM) with Artificial Neural Network (ANN) in lipase-catalyzed synthesis of palm-based wax ester. BMC Biotechnol., 7: 53-63.
CrossRef PMid:17760990 PMCid:PMC2211475
-
Baxt, W.G., 1991. Use of an artificial neural network for the diagnosis of myocardial infarction. Ann. Int. Med., 115: 843-848.
CrossRef PMid:1952470
-
Bourquin, J., H. Schmidli, P.V. Hoogevest, A. Leu-Enberger, 1998. Advantages of Artificial Neural Networks (ANNs) as alternative modeling technique for data sets showing non-linear relationships using data from a galenical study on a solid dosage form. Eur. J. Pharm. Sci., 7(1): 15-16.
CrossRef
-
Dayhoff, J.E. and J.M. DeLeo, 2001. Artificial neural networks. Cancer, 91: 1615-35.
CrossRef
-
Deng, N., Y. Tian and C. Zhang, 2012. Support Vector Machines: Optimization Based Theory, Algorithms and Extensions. 1st Edn., Chapman and Hall/CRC, New York.
-
Desai, K.M., S.A. Survase, P.S. Saudagar, S.S. Lele and R.S. Singhal, 2008. Comparison of Artificial Neural Network (ANN) and Response Surface Methodology (RSM) in fermentation media optimization: Case study of fermentative production of scleroglucan. Biochem. Eng. J., 41(3): 266-273.
CrossRef
-
Fan, R.E., K.W. Chang, C.J. Hsieh, X.R. Wang and C.J. Lin, 2008. LIBLINEAR: A library for large linear classification. J. Mach. Learn. Res., 9: 1871-1874.
-
Friesen, D.D., M. Patterson and B. Harmel, 2011. A comparison of multiple regression andneural networks for forecasting real estate values. Reg. Bus. Rev., 30: 114-36.
-
Goulermas, J.Y., P. Liatsis, X.J. Zeng and P. Cook, 2007. Density-Driven Generalized Regression Neural Networks (DD-GRNN) for function approximation. IEEE T. Neural Networ., 18: 1683-1696.
CrossRef PMid:18051185
-
Guo, Q. and Y. Liu, 2010. ModEco: An integrated software package for ecological niche modeling. Ecography, 33: 637-642.
CrossRef
-
Hao, J.Y., W. Han, S. Huang, B. Xue and X. Deng, 2000. Microwave assisted extraction of artemisinin from Artemisiaannua L. Sep. Purif. Technol., 28(3): 191-196.
CrossRef
-
Hopfield, J.J., 1988. Artificial neural networks. IEEE Circuit Devic., 4: 3-10.
CrossRef
-
Hoskins, J.C. and D.M. Himmelblau, 1998. Artificial neural network models of knowledge representation in chemical engineering. Comput. Chem. Eng., 12: 881-890.
CrossRef
-
Kandirmaz, H.M., K. Kaba and M. Avci, 2014. Estimation of monthly sunshine duration in Turkey using artificial neural networks. Int. J. Photoenerg., 2014: 1-9.
CrossRef
-
Khan, J., J.S. Wei, M. Ringné, L.H. Saal, M. Ladanyi, F. Westermann et al., 2007. Classification and diagnostic prediction of cancers using gene expression profiling and artificial neural networks. Nat. Med., 7: 673-679.
CrossRef PMid:11385503 PMCid:PMC1282521 -
Kim, D.W., K. Lee, D. Lee and K.H. Lee, 2005. A kernel-based subtractive clustering method. Pattern Recogn. Lett., 26: 879-891.
CrossRef -
Xu and X. Liu, 2014. Prediction of Henry's law constants for organic compounds using multilayer feed for ward neural networks based on linear solvation energy relationship. J. Chem. Pharm. Res., 6(6): 1557-1564.
-
Li, H., W. Leng, Y. Zhou, F. Chen, Z. Xiu and D. Yang, 2014. Evaluation models for soil nutrient based on support vector machine and artificial neural networks. Sci. World J., 2014: 478569.
CrossRef -
Lou, W. and S. Nakai, 2001. Application of artificial neural networks for predicting the thermal inactivation of bacteria: A combined effect of temperature, pH and water activity. Food Res. Int., 34(7): 573-591.
CrossRef
-
Manohar, B. and S. Divakar, 2005. An artificial neural network analysis of porcine pancreas lipase catalysed esterification of anthranilic acid with methanol. Process Biochem., 40(10): 3372-3376.
CrossRef -
Mansour, G.M. and K. Mostafa, 2011. Comparison of response surface methodology and artificial neural network in predicting the microwave-assisted extraction procedure to determine zinc in fish muscles. Food Nutr. Sci., 2: 803-808.
CrossRef -
Montgomery, D.C., 2004. Design and Analysis of Experiments. Wiley, Hoboken.
-
Pareek, V.K., M.P. Brungs, A.A. Adesina and R. Sharma, 2002. Artificial neural network modeling of a multiphase photodegradation system. J. Photoch. Photobio. A, 149: 139-146.
CrossRef
-
Pollar, M., M. Jaroensutasinee and K. Jaroensutasinee, 2007. Morphometric analysis of Tor tambroides by stepwise discriminant and neural network analysis. World Acad. Sci. Eng. Technol., 33: 16-20.
-
Sharma, P., L. Singh and N. Dilbaghi, 2008. Optimization of process variables for decolorization of disperse yellow 211 by bacillus subtilis using box-behnken design. J. Hazard. Mater., 164: 2-3.
CrossRef PMid:18845394
-
Shen, Y., Z. He, Q. Wang and Y. Wang, 2012. Feature generation of hyperspectral images for fuzzy support vector machine classification. Proceeding of the IEEE International Instrumentation and Measurement Technology Conference (I2MTC), Graz, pp: 1977-1982.
CrossRef -
Specht, D.F., 1991. A general regression neural network. IEEE T. Neural. Netw., 2: 568-576.
CrossRef PMid:18282872 -
Svozil, D., V. Kvasnickab and J. Pospichalb, 1997. Introduction to multi-layer feed-forward neural networks. Chemom. Intell. Lab. Syst., 39: 43-62.
CrossRef -
Vouk, D., D. Malus and I. Halkijevic, 2011. Neural networks in economic analyses of wastewater systems. Expert Syst. Appl., 38: 10031-10035.
CrossRef
-
Yang, D., H. Li, C. Cao, F. Chen, Y. Zhou and Z. Xiu, 2014. Analysis of the oil content of rapeseed using artificial neural networks based on near infrared spectral data. J. Spectroscopy., 2014.
CrossRef -
Yegnanarayana, B., 2009. Artificial Neural Networks. Prentice-Hall of India, New Delhi.
PMid:19813815 -
Zhong, X., J. Li, H. Dou, S.J. Deng, G.F. Wang, Y. Jiang et al., 2013. Fuzzy nonlinear proximal support vector machine for land extraction based on remote sensing image. PLoS One, 8: e69434.
CrossRef PMid:23936016 PMCid:PMC3720649
Competing interests
The authors have no competing interests.
Open Access Policy
This article is distributed under the terms of the Creative Commons Attribution 4.0 International License (http://creativecommons.org/licenses/by/4.0/), which permits unrestricted use, distribution, and reproduction in any medium, provided you give appropriate credit to the original author(s) and the source, provide a link to the Creative Commons license, and indicate if changes were made.
Copyright
The authors have no competing interests.
|
|
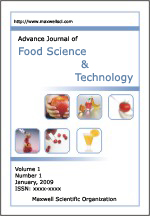 |
ISSN (Online): 2042-4876
ISSN (Print): 2042-4868 |
 |
Information |
|
|
|
Sales & Services |
|
|
|