Research Article | OPEN ACCESS
A Multi-industry Default Prediction Model using Logistic Regression and Decision Tree
1Suresh Ramakrishnan, 1Maryam Mirzaei and 2Mahmoud Bekri
1Faculty of Management, Universiti Teknologi Malaysia, Malaysia
2Economic and Statistic Institute, Karlsruhe Institute of Technology, Germany
Research Journal of Applied Sciences, Engineering and Technology 2015 10:856-861
Received: November ‎19, ‎2014 | Accepted: January ‎8, ‎2015 | Published: April 05, 2015
Abstract
The accurate prediction of corporate bankruptcy for the firms in different industries is of a great concern to investors and creditors, as the reduction of creditors’ risk and a considerable amount of saving for an industry economy can be possible. Financial statements vary between industries. Therefore, economic intuition suggests that industry effects should be an important component in bankruptcy prediction. This study attempts to detail the characteristics of each industry using sector indicators. The results show significant relationship between probability of default and sector indicators. The results of this study may improve the default prediction models performance and reduce the costs of risk management.
Keywords:
Decision tree, default prediction, industry effects , logistic regression,
Competing interests
The authors have no competing interests.
Open Access Policy
This article is distributed under the terms of the Creative Commons Attribution 4.0 International License (http://creativecommons.org/licenses/by/4.0/), which permits unrestricted use, distribution, and reproduction in any medium, provided you give appropriate credit to the original author(s) and the source, provide a link to the Creative Commons license, and indicate if changes were made.
Copyright
The authors have no competing interests.
|
|
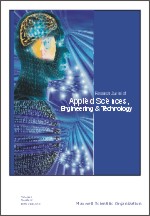 |
ISSN (Online): 2040-7467
ISSN (Print): 2040-7459 |
 |
Information |
|
|
|
Sales & Services |
|
|
|