Research Article | OPEN ACCESS
A Hybrid Feature Subset Selection using Metrics and Forward Selection
1K. Fathima Bibi and 2M. Nazreen Banu
1Department of Computer Science, Bharathiar University, Coimbatore, TN, India
2Department of MCA, MAM College of Engineering, Tiruchirappalli, TN, India
Research Journal of Applied Sciences, Engineering and Technology 2015 10:834-840
Received: November 10, 2014 | Accepted: January 27, 2015 | Published: April 05, 2015
Abstract
The aim of this study is to design a Feature Subset Selection Technique that speeds up the Feature Selection (FS) process in high dimensional datasets with reduced computational cost and great efficiency. FS has become the focus of much research on decision support system areas for which data with tremendous number of variables are analyzed. Filters and wrappers are proposed techniques for the feature subset selection process. Filters make use of association based approach but wrappers adopt classification algorithms to identify important features. Filter method lacks the ability of minimization of simplification error while wrapper method burden weighty computational resource. To pull through these difficulties, a hybrid approach is proposed combining both filters and wrappers. Filter approach uses a permutation of ranker search methods and a wrapper which improves the learning accurateness and obtains a lessening in the memory requirements and finishing time. The UCI machine learning repository was chosen to experiment the approach. The classification accuracy resulted from our approach proves to be higher.
Keywords:
Algorithm, filter , machine learning , ranker search, repository, wrapper,
Competing interests
The authors have no competing interests.
Open Access Policy
This article is distributed under the terms of the Creative Commons Attribution 4.0 International License (http://creativecommons.org/licenses/by/4.0/), which permits unrestricted use, distribution, and reproduction in any medium, provided you give appropriate credit to the original author(s) and the source, provide a link to the Creative Commons license, and indicate if changes were made.
Copyright
The authors have no competing interests.
|
|
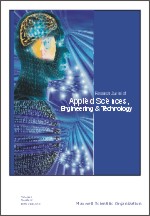 |
ISSN (Online): 2040-7467
ISSN (Print): 2040-7459 |
 |
Information |
|
|
|
Sales & Services |
|
|
|