Research Article | OPEN ACCESS
Agent-based Modeling in Supply Chain Management using Improved C4-5
1C.P. Balasubramaniam and 2V. Thigarasu
1Karpagam University, Coimbatore, Tamil Nadu 641021
2Depertament of Computer Science, Gobi Arts and Science College, Gobichettipalayam, India
Research Journal of Applied Sciences, Engineering and Technology 2015 2:91-97
Received: August ‎13, ‎2014 | Accepted: September ‎14, ‎2014 | Published: January 15, 2015
Abstract
The Supply chain can describe the activities that are involved in the chain, or the companies, or the different functions. In literature there are a lot of models describing the Supply chain from different perspectives. Currently supply chains performance measurement systems suffer from being too inward looking, ignoring external environmental factors that might affect the overall supply chain performance when setting new targets. The most efficient Supply chain is the one that has the lowest possible cost and at the same time meet the customer’s expectations on service like delivery precision and lead-time. In this study decision based technique C4.5 is improved using correlation coefficient of Kendall for effective classification. The correlation coefficient of Kendall is adapted to improve the system. The C4.5 not only produce discrete attributes, but also continuous ones can be handled, handling incomplete training data with missing values and it is prune during the construction of trees to avoid over-fitting. The accuracy is calculated by sensitivity and specificity for the proposed and existing technique for the textile synthesis dataset. Obtained results will prove the efficiency of this proposed technique based on its accuracy.
Keywords:
Decision trees , fuzzy classification , Kendall, supply chain,
References
- Anderson, P., H. Aronson and N.G. Storhagen, 1989. Measuring logistics performance. Eng. Cost. Prod. Econ., 17: 253-262.
CrossRef - Chandra, C. and A. Tumanyan, 2007. Organization and problem ontology for supply chain information support system. Data Knowl. Eng., 61(2): 263-280.
CrossRef
-
Chen, I.J. and A. Paulraj, 2004. Towards a theory of supply chain management: The constructs and measurements. J. Oper. Manag., 22(2): 119-150.
CrossRef
-
Christopher, M., 1998. Logistics and Supply Chain Management: Strategies for Reducing Costs and Improving Services. 2nd Edn., Pitman, London.
PMCid:PMC1565158
-
Cooper, M.C., D.M. Lambert and J.D. Pagh, 1997. Supply chain management: More than a new name for logistics. Int. J. Logist. Manage., 8(1): 1-14.
CrossRef
-
Croom, S., P. Romano and M. Giannakis, 2000. Supply chain management: An analytical framework for critical literature review. Eur. J. Purchas. Supply Manage., 6(1): 67-83.
CrossRef
-
Estampe, D., S. Lamouri, J.L. Paris and S. Brahim-Djelloul, 2013. A framework for analysing supply chain performance evaluation models. Int. J. Prod. Econ., 142(2): 247-258.
CrossRef
- Ganga, G.M.D. and L.C.R. Carpinetti, 2011. A fuzzy logic approach to supply chain performance management. Int. J. Prod. Econ., 134(1): 177-187.
CrossRef
- Gosain, S., A. Malhotra and O.A. El Sawy, 2004. Coordinating for flexibility in e-business supply chains. J. Manage. Inform. Syst., 21(3): 7-45.
- Guarino, N., 1998. Formal ontology and information systems. Proceeding of 1st International Conference on Formal Ontology (FOIS, 1998), Trento, Italy.
- Gunasekaran, A. and E.W.T. Ngai, 2004. Information systems in supply chain integration and management. Eur. J. Oper. Res., 159(2): 269-295.
CrossRef
-
Huan, S.H., S.K. Sheoran and G. Wang, 2004. A review and analysis of Supply chain operations reference (SCOR) model. Supply Chain Manag., 9(1): 23-29.
CrossRef
- Hult, G.T.M., D.J. Ketchen, S.T. Cavusgil and R.J. Calantone, 2006. Knowledge as a strategic resource in supply chains. J. Oper. Manag., 24(5): 458-475.
CrossRef
-
Higginson, J.K. and A. Alam, 1997. Supply chain management techniques in medium-to-small manufacturing firms. Int. J. Logist. Manage., 8(2): 19-32.
CrossRef
- Jain, V., S. Wadhwa and S.G. Deshmukh, 2009. Revisiting information systems to support a dynamic supply chain: Issues and perspectives. Prod. Plan. Control Manage. Oper., 20(1): 17-29.
CrossRef
-
Kendall, M.G., 1955. Rank Correlation Methods. Hafner Publishing Co., New York.
- Kishore, R., R. Sharman and R. Ramesh, 2004. Computational ontologies and information systems I: Foundations. Communi. Associat. Inform. Syst., 14(Article 8): 158-183.
- Lambert, D.M. and M.C. Cooper, 2000. Issues in supply chain management. Ind. Market. Manag., 29(1): 65-83.
CrossRef
- Murthy, S.K., 1998. Automatic construction of decision trees from data: A multi-disciplinary survey. Data Min. Knowl. Disc., 2: 345-389.
CrossRef
- Norman, M.S., D.W. Hildum and D. Kjenstad, 2003. Agent-based e-supply chain decision support. J. Org. Comp. Elect. Com., 13(3-4): 225-241.
-
Paulraj, A., A. Lado and I.J. Chen, 2008. Inter-organizational communication as a relational competency: Antecedents and performance outcomes in collaborative buyer- supplier relationships. J. Oper. Manag., 26(1): 45-64.
CrossRef
- Quinlan, J.R., 1979. Discovering Rules by Induction from Large Collections of Examples. In: Michie, D. (Ed.), Expert Systems in the Microelectronic Age. Edinburg University Press, Edinburg, pp: 168-201.
- Quinlan, J.R., 1993. C4.5: Programs for Machine Learning. Morgan Kaufmann, San Francisco.
PMCid:PMC1554917
- Rai, A., R. Patnayakuni and N. Seth, 2006. Firm performance impacts of digitally enabled supply chain integration capabilities. MIS Quart., 30(2): 225-246.
CrossRef
- Selwyn, P., 2005. Machine learning for dynamic multi-product supply chain formation. Expert Syst. Appl., 29(4): 985-990.
CrossRef
-
Solvang, W.D., 2001. Architecture for supply chain analysis and methodology for quantitative measurement of supply chain flexibility. Ph.D. Thesis, Norwegian University of Science and Technology, Trondheim.
- Sotiris, K. and P. Panayotis, 2009. Predictive Data Mining: A Survey of Regression Methods. In: Khosrow-Pour, M. (Ed.), the Encyclopedia of Information Science and Technology. 2nd Edn., 6: 3105-3110.
- Sotiris, Z., 2000. Supply chain management. Report Produced for the EC Funded Project INNOREGIO: Dissemination of Innovation and Knowledge Management Techniques, BPR Hellas, 2000.
-
Stainer, A., 1997. Logistics-a productivity and performance perspective. Supply Chain Manag., 2(2): 53-62.
CrossRef
- Svensson, G., 2003. Holistic and cross-disciplinary deficiencies in the theory generation of Supply chain management. Supply Chain Manag., 8(4): 303-316.
CrossRef
- Sycara, K.P., 1998. Multiagent systems. AI Mag., 19(2): 79-92.
-
Tummala, V.M.R., C.L.M. Phillips and M. Johnson, 2006. Assessing supply chain management success factors: A case study. Supply Chain Manag., 11(2): 179-192.
CrossRef
-
Wooldridge, M., 1999. Intelligent Agents. In: Gerhard, W. (Ed.), Multiagent Systems: A Modern Approach to Distribute Artificial Intelligence. The MIT Press, Cambridge, 1: 27-78.
- Zdravkovic, M., H. Panetto, M. Trajanovic and A. Aubry, 2011. An approach for formalizing the supply chain operations. Lect. Notes Bus. Inf., 5(4): 401-421.
Competing interests
The authors have no competing interests.
Open Access Policy
This article is distributed under the terms of the Creative Commons Attribution 4.0 International License (http://creativecommons.org/licenses/by/4.0/), which permits unrestricted use, distribution, and reproduction in any medium, provided you give appropriate credit to the original author(s) and the source, provide a link to the Creative Commons license, and indicate if changes were made.
Copyright
The authors have no competing interests.
|
|
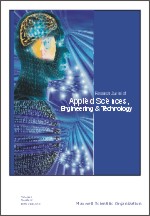 |
ISSN (Online): 2040-7467
ISSN (Print): 2040-7459 |
 |
Information |
|
|
|
Sales & Services |
|
|
|