Research Article | OPEN ACCESS
Ant Colony Optimization: A Review and Comparison
Sundus Shaukat, Riaz Ahmed Bhatti, Khalid Ibrahim Qureshi and Shafqat Ali Shad
Department of Computer Science, COMSATS Institute of Information Technology, Wah Cantt, Pakistan
Research Journal of Applied Sciences, Engineering and Technology 2014 3:435-438
Received: April 15, 2014 | Accepted: May 24, 2014 | Published: July 15, 2014
Abstract
Many optmization algorithms are developed over period of time, among these most famous and widely used is Ant Colony systems (ACA). Ant Colony Systems (ACS) are the collection of different ant colony optimization algorithms. Different algorithms are used for solve the Travelling salesmen Problem (TCP) but ant colony algorithm is more preferred to solve the travelling salesmen problem. In ant colony best solution is found with the help of cooperating agents called ants. Ants cooperate with each other and communicate using an indirect way with the help of pheromone which is deposited on the edges on the path. To achieve the finest solution, different ant colony techniques are compared. In this study we compared different Ant Colony Systems (ACS) algorithms through their implementation on classical problem of Travelling salesmen Problem (TCP).
Keywords:
Ant colony algorithm optimization, neural networks,
References
-
Afshar, M.H., 2009. Elitist-mutated ant system versus max-min ant system: Application to pipe network optimization problems. T. A Civil Eng., 16(4): 286-296.
-
Balaprakash, P., M. Birattari, T. Stutzle, Z. Yuan and M. Dorigo, 2009. Estimation-based ant colony optimization and local search for the probabilistic traveling salesman problem. Swarm Intell., 3(3): 223-242.
CrossRef -
Bullnheimer, B., R.F. Hartl and C. Strauss, 1997. A new rank based version of the ant system: A computational study. Cent. Europ. J. Oper. Re. Econ., 7: 25-38.
-
Chu, S., J. Roddick and J. Pan, 2004. Ant colony system with communication strategies. Inform. Sci., 167(1): 63-76.
CrossRef -
Deneubourg, J., S. Aron, S. Goss and J. Pasteels, 1990. The self-organizing exploratory pattern of the argentine ant. J. Insect Behav., 3(2): 159-168.
CrossRef -
Dorigo, M., 1992. Optimization, learning and natural algorithms. Ph.D. Thesis, Politecnico Di Milano, Italy.
-
Dorigo, M. and L. Gambardella, 1997. Ant colony system: A cooperative learning approach to the traveling salesman problem. IEEE T. Evolut. Comput., 1(1): 53-66.
CrossRef -
Dorigo, M. and T. Stutzle, 2003. The Ant Colony Optimization Metaheuristic: Algorithms, Applications and Advances. Handbook of Metaheuristics. Springer, Heidelberg, 57: 250-285.
-
Dorigo, M., V. Maniezzo and A. Colorni, 1996. Ant system: Optimization by a colony of cooperating agents. IEEE T. Syst. Man Cy. B, 26(1): 29-41.
CrossRef PMid:18263004 -
Dorigo, M., M. Birattari and T. Stutzle, 2006. Ant colony optimization. IEEE Comput. Intell. M., 1(4): 28-39.
CrossRef -
Gambardella, L. and M. Dorigo, 1995. Ant-Q: A reinforcement learning approach to the traveling salesman problem. Proceeding of ML-95 the 12th International Conference on Machine Learning. Morgan Kaufmann, pp: 252-260.
CrossRef -
Goss, S., S. Aron, J. Deneubourg and J. Pasteels, 1989. Self-organized shortcuts in the Argentine ant. Naturwissenschaften, 76(12): 579-581.
CrossRef -
Graupe, D. and R. Gandhi, 2001. Implementation of traveling salesman's problem using neural network. Final Project Report (Fall 2001), ECE 559 Neural Networks, pp: 1-9.
-
Hammerl, T., 2009. Ant colony optimization for tree and hypertree decompositions. M.A. Thesis, Vienna University of Technology.
-
Holldobler, B., M. Obermayer and E. Wilson, 1992. Communication in the primitive cryptobiotic ant Prionopelta amabilis (Hymenoptera: Formicidae). J. Comp. Physiol. A, 171(1): 9-16.
CrossRef -
Ilie, S. and C. Badica, 2010. Effetiveness of solving traveling salesman problem using ant colony optimization on distributed multi-agent middleware. Proceeding of the International Multiconference on Computer Science and Information Technology (IMCSIT, 2010), pp: 197-203.
-
Korb, O., T. Stützle and T.E. Exner, 2006. PLANTS: Application of ant colony optimization to structure-based drug design. In: Dorigo, M., L.M. Gambardella, M. Birattari, A. Martinoli, R. Poli and T. Stützle (Eds.), Ant Colony Optimization and Swarm Intelligence, 5th International Workshop, ANTS 2006, LNCS 4150, pp: 247-258.
-
Manyppt, 2014. (Assecced on: May 19, 2014).
Direct Link -
Negulescu, S., C. Oprean, C. Kifor and I. Carabulea, 2008. Elitist ant system for route allocation problem. Proceedings of the 8th WSEAS International Conference on Applied Informatics and Communications (AIC'08).
PMCid:PMC2650422 -
Stutzle, T. and H. Hoos, 2000. MAX--MIN ant system. Future Gener. Comp. Sy., 16(8): 889-914.
CrossRef
Competing interests
The authors have no competing interests.
Open Access Policy
This article is distributed under the terms of the Creative Commons Attribution 4.0 International License (http://creativecommons.org/licenses/by/4.0/), which permits unrestricted use, distribution, and reproduction in any medium, provided you give appropriate credit to the original author(s) and the source, provide a link to the Creative Commons license, and indicate if changes were made.
Copyright
The authors have no competing interests.
|
|
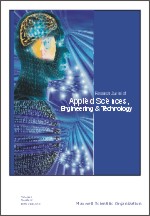 |
ISSN (Online): 2040-7467
ISSN (Print): 2040-7459 |
 |
Information |
|
|
|
Sales & Services |
|
|
|