Research Article | OPEN ACCESS
Sparsity-constraint LMS Algorithms for Time-varying UWB Channel Estimation
1, 2Solomon Nunoo, 1Uche A.K. Chude-okonkwo and 1Razali Ngah
1Wireless Communication Centre, Universiti Teknologi Malaysia, Johor, Malaysia
2Department of Electrical and Electronic Engineering, University of Mines and Technology, Tarkwa, Ghana
Research Journal of Applied Sciences, Engineering and Technology 2014 24:2408-2415
Received: August 03, 2014 | Accepted: September 14, 2014 | Published: December 25, 2014
Abstract
Sparsity constraint channel estimation using compressive sensing approach has gained widespread interest in recent times. Mostly, the approach utilizes either the l1-norm or l0-norm relaxation to improve the performance of LMS-type algorithms. In this study, we present the adaptive channel estimation of time-varying ultra wideband channels, which have shown to be sparse, in an indoor environment using sparsity-constraint LMS and NLMS algorithms for different sparsity measures. For a less sparse CIR, higher weightings are allocated to the sparse penalty term. Simulation results show improved performance of the sparsity-constraint algorithms in terms of convergence speed and mean square error performance.
Keywords:
Compressive sensing, (N) LMS algorithms, sparse channel estimation, time-varying channels, ultra wideband,
References
-
Chen, Y., Y. Gu and A.O. Hero, 2009. Sparse LMS for system identification. Proceeding of IEEE International Conference on Acoustics, Speech and Signal Processing. Taipei, pp: 3125-3128.
-
Das, B.K., M. Chakraborty and S. Banerjee, 2011. Adaptive identification of sparse systems with variable sparsity. Proceeding of IEEE International Symposium of Circuits and Systems (ISCAS, 2011). Rio de Janeiro, Brazil, pp: 1267-1270.
CrossRef -
Diniz, P.S.R., 2013. Adaptive Filtering: Algorithms and Practical Implementation. 4th Edn., Springer, New York.
CrossRef -
Donoho, D.L., 2006. Compressed sensing. IEEE T. Inform. Theory, 52(4): 1289-1306.
CrossRef -
Gu, Y., J. Jin and S. Mei, 2009. l0 norm constraint LMS algorithm for sparse system identification. IEEE Signal Proc. Let., 16(9): 774-777.
CrossRef -
Gui, G. and F. Adachi, 2013. Improved least mean square algorithm with application to adaptive sparse channel estimation. EURASIP J. Wirel. Comm., 2013(1): 204.
-
Gui, G., W. Peng and F. Adachi, 2013b. Adaptive system identification using robust LMS/F algorithm. Int. J. Commun. Syst., 27(11): 2956-2963.
CrossRef -
Gui, G., S. Kumagai, A. Mehbodniya and F. Adachi, 2013a. Variable is good: Adaptive sparse channel estimation using VSS-ZA-NLMS algorithm. Proceeding of 2013 IEEE International Conference on Wireless Communications and Signal Processing, pp: 1-5.
CrossRef PMid:24060924 -
Haykin, S., 2002. Adaptive Filter Theory. 4th Edn., Prentice-Hall, Inc., New Jersey.
PMid:12487794 -
Kaiser, T. and F. Zheng, 2010. Ultra Wideband Systems with MIMO. John Wiley and Sons, Ltd., Chichester, UK.
CrossRef -
Kaiser, T., F. Zheng and E. Dimitrov, 2009. An overview of ultra-wide-band systems with MIMO. P. IEEE, 97(2): 285-312.
CrossRef -
Khong, A.W.H. and P.A. Naylor, 2006. Efficient use of sparse adaptive filters. Proceeding of IEEE 2006 Fortieth Asilomar Conference on Signals, Systems and Computers. Pacific Grove, CA, pp: 1375-1379.
CrossRef -
Li, Y. and M. Hamamura, 2014. An improved proportionate normalized least-mean-square algorithm for broadband multipath channel estimation. Sci. World J., 2014(2014): 9, Article ID 572969.
-
Molisch, A.F., 2005. Ultrawideband propagation channels-theory, measurement and modeling. IEEE T. Veh. Technol., 54(5): 1528-1545.
CrossRef -
Sayed, A.H., 2008. Adaptive Filters. John Wiley and Sons, Inc., Hoboken, NJ, USA.
CrossRef -
Taheri, O. and S.A. Vorobyov, 2011. Sparse channel estimation with lp-norm and reweighted l1-norm penalized least mean squares. Proceeding of IEEE International Conference on Acoustics, Speech and Signal Processing (ICASSP, 2011). Prague, Czech Republic, pp: 2864-2867.
-
Taheri, O. and S.A.Vorobyov, 2014. Reweighted l1-norm penalized LMS for sparse channel estimation and its analysis. Signal Process., 104: 70-79.
CrossRef
Competing interests
The authors have no competing interests.
Open Access Policy
This article is distributed under the terms of the Creative Commons Attribution 4.0 International License (http://creativecommons.org/licenses/by/4.0/), which permits unrestricted use, distribution, and reproduction in any medium, provided you give appropriate credit to the original author(s) and the source, provide a link to the Creative Commons license, and indicate if changes were made.
Copyright
The authors have no competing interests.
|
|
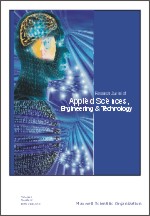 |
ISSN (Online): 2040-7467
ISSN (Print): 2040-7459 |
 |
Information |
|
|
|
Sales & Services |
|
|
|