Research Article | OPEN ACCESS
Computer Assisted Diagnosis of Brain Tumor in MRI Images using Texture Features as Input to Ada-boost Classifier
1A. Prabin and 2J. Veerappan
1Department of ECE, Universal College of Engineering and Technology
2Department of ECE, Sethu Institute of Technology, Tamilnadu, India
Research Journal of Applied Sciences, Engineering and Technology 2014 24:2374-2380
Received: March 14, 2014 | Accepted: September 04, 2014 | Published: December 25, 2014
Abstract
In medical image processing, segmentation is an important and challenging task. It is classically used to identify object contours and extract the object from the image. Tumor Classification is an significant in medical image analysis since it provides information related to anatomical structures as well as possible anomalous tissues necessary to treatment planning and patient follow-up. In this study, a new approach for automatic classification of brain tumor in enhanced MRI images is developed. Our proposed method consists of Five steps: i) Preprocessing ii) Tumor Region Segmentation iii) Feature Extraction using Wavelet and Level set method iv) Feature Selection and v) Feature Classification using Ada-Boost classifier. The experimental results are validated using the evaluation metrics such as sensitivity, specificity and accuracy. Our proposed system experimental results are compared to other neural network based classifier such as Feed Forward Neural Network (FFNN) and Radial Basics Function (RBF). The classification accuracy of proposed method produces better results compared to other leading tumor classification methods.
Keywords:
Classification, DWT, feature extraction, MRI, PCA, segmentation, tumor,
References
-
Demirkaya, O., 2002. Anisotropic diffusion filtering of PET attenuation data to improve emission images. Phys. Med. Biol., 47: 271-278.
CrossRef -
Freund, Y. and R. Schapire, 1994. A decision-theoretic generalization of on-line learning and an application to boosting. Proceeding of European Conference on Computational Learning Theory, LNCS (EuroCOLT'94).
-
Gonzalez, R.C. and R.E. Woods, 2004. Digital image processing. 2nd Edn., Pearson Education, New Delhi.
-
Guyon, I. and A. Elisseeff, 2003. An introduction to variable and feature selection. J. Mach. Learn. Res., 3: 1157-1182.
-
Hiremath, P.S., S. Shivashankar and P. Jagadeesh, 2006. Wavelet based features for color texture classification with application to CBIR. Int. J. Comput. Sci. Network Sec., 6(9A): 124-133.
-
Jayachandran, A. and R. Dhanasekaran, 2013a. Automatic detection of brain tumor in magnetic resonance images using multi-texton histogram and support vector machine. Int. J. Imag. Syst. Tech., 23: 97-103.
CrossRef -
Jayachandran, A. and R. Dhanasekaran, 2013b. Brain tumor detection using fuzzy support vector machine classification based on a texton co-occurrence matrix. J. Imaging Sci. Techn., 57(1): 10507-1-10507-7(7).
-
Kidwell, C.S. and M. Wintermark, 2010. The role of CT and MRI in the emergency evaluation of persons with suspected stroke. Curr. Neurol. Neurosci., 10(1): 21-28.
CrossRef PMid:20425222 -
Lia, B., Q. Yuan, Z. Liu, X. Li and X. Yin, 2008. Automatic segmentation of intracranial hematoma and volume measurement. Proceeding of 30th Annual International Conference of the IEEE Engineering in Medicine and Biology Society, (EMBS, 2008), pp: 1214-1217.
CrossRef -
Novovicova, J., P. Pudil and J. Kittler, 1996. Divergence based feature selection for multimodal class densities. IEEE T. Pattern Anal., 18(2): 218-223.
CrossRef -
Osher, S. and J.A. Sethian, 1988. Fronts propagating with curvature-dependent speed: Algorithms based on Hamilton-Jacobi formulations. J. Comput. Phys., 79: 12-49.
CrossRef -
Roy, S. and S.K. Bandyopadhyay, 2012. Detection and quantification of brain tumor from MRI of brain and it's symmetric analysis. Int. J. Inform. Commun. Technol. Res., 2(6): 2223-4985.
-
Schapire, R.E., 1999. A brief introduction to boosting. Proceeding of the 16th International Joint Conference on Artificial Intelligence, Morgan Kaufmann Publishers Inc. San Francisco, CA, USA, 2: 1401-1406.
-
Sengur, A., 2008. An expert system based on principal component analysis, artificial immune system and fuzzy k-NN for diagnosis of valvular heart diseases. Comput. Biol. Med., 51(3): 329-338.
CrossRef PMid:18177849 -
Suri, S., K. Liu, S. Singh, S.N. Laxminarayan, X. Zeng and L. Reden, 2002. Shape recovery algorithms using level sets in 2-D/3-D medical imagery: A state-of-the-art review. IEEE T. Inf. Technol. B., 6(1): 8-28.
CrossRef -
Weisenfield, N.I. and S.K. Warfield, 2004. Normalization of joint image-intensity statistics in MRI using the Kullback-Leibler divergence. Proceeding of IEEE International Symposium Biomedical Imaging: Nano to Macro, 1: 101-104.
CrossRef -
Zhu, W., N. Zeng and N. Wang, 2010. Sensitivity, specificity, accuracy, associated confidence interval and ROC analysis with practical SAS implementations. Proceeding of the SAS Conference. Baltimore, Maryland.
-
Zhu, G., S. Zhang, Q. Zeng and C. Wang, 2007. Boundary-based image segmentation using binary level set method. Opt. Eng., 46: 050501.
CrossRef
Competing interests
The authors have no competing interests.
Open Access Policy
This article is distributed under the terms of the Creative Commons Attribution 4.0 International License (http://creativecommons.org/licenses/by/4.0/), which permits unrestricted use, distribution, and reproduction in any medium, provided you give appropriate credit to the original author(s) and the source, provide a link to the Creative Commons license, and indicate if changes were made.
Copyright
The authors have no competing interests.
|
|
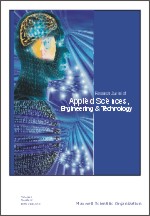 |
ISSN (Online): 2040-7467
ISSN (Print): 2040-7459 |
 |
Information |
|
|
|
Sales & Services |
|
|
|