Research Article | OPEN ACCESS
Modified Structural and Attribute Clustering Algorithm for Improving Cluster Quality in Data Mining: A Quality Oriented Approach
1G. Abel Thangaraja and 2Saravanan Venkataraman Tirumalai
1Department of Computer Science, Kaypeeyes College of Arts and Science, Kotagiri, the Nilgiris, India
2College of Computer and Information Sciences, Majmaah University, Majmaah,
Kingdom of Saudi Arabia
Research Journal of Applied Sciences, Engineering and Technology 2014 20:2097-2102
Received: June 08, 2014 | Accepted: July 13, 2014 | Published: November 25, 2014
Abstract
The need of Data mining is because of the explosive growth of data from terabytes to petabytes. Data mining preprocess aims to produce the quality mining result in descriptive and predictive analysis. The quality of a clustering result depends on both the similarity measure used by the method and its implementation. A straightforward way to combine structural and attribute similarities is to use a weighted distance function. Clustering results are arrived based on attribute similarities. The clusters balance the attribute and structural similarities. The existing Structural and Attribute cluster algorithm is analyzed and a new algorithm is proposed. Both the algorithms are compared and results are analyzed. It is found that the modified algorithm gives better quality clusters.
Keywords:
Attribute similarity, cluster quality, data mining, structural,
References
-
Cheng, H., Y. Zhou and J.X. Yu, 2011. Clustering large attributed graphs: A balance between structural and attribute similarities. ACM T. Knowl. Discov. Data, 5(2), Article12.
-
Ghasemi, M., M. Rahgozar, G. Bidkhori and A. Masoudi-Nejad, 2013. C-element: A new clustering algorithm to find high quality functional modules in PPI networks. PLoS one 8(9), DOI: 10.1371/journal.pone.0072366.
CrossRef
-
Han, J., M. Kamber and J. Pei, 2011. Data Mining: Concepts and Techniques. 3rd Edn., Elsevier Science, Burlington.
Direct Link
-
Jayabrabu, R., V. Saravanan and K. Vivekanandan, 2012. A framework: Cluster detection and multidimensional visualization of automated data mining using intelligent agents. Int. J. Artif. Intell. Appl., 3(1): 15.
CrossRef
-
Jeyabalaraja, V. and T. Edwin Prabakaran, 2012. Study on software process metrics using data mining tool-A rough set theory approach. Int. J. Comput. Appl., 47(18): 0975-888.
-
Orme, B. and R. Johnson, 2008. Improving K-means cluster analysis: ensemble analysis instead of highest reproducibility replicates. Proceeding of the Saw Tooth Software Conference. Sequim WA.
-
Pons, P. and M. Latapy, 2006. Computing communities in large networks using random walks. J. Graph Algorithm. Appl., 10(2): 191-218.
-
Rai, P. and S. Singh, 2005. A survey of clustering techniques. Int. J. Comput. Appl., 7(12): 1-5.
-
Strehl, A. and J. Ghosh, 2002. Clustering ensembles-A knowledge reuse framework for combining multiple partitions. J. Mach. Learn. Res., 3(2002): 583-617.
-
Tajunisha, N. and V. Saravanan, 2011. A new approach to improve the clustering accuracy using informative genes for unsupervised microarray data sets. Int. J. Adv. Sci. Technol., 27: 85-94.
-
Tsai, C.Y. and C.C. Chui, 2008. Developing a feature weight self-adjustment mechanism for a K-means clustering algorithm. Comput. Stat. Data An., 52: 4658-4672.
CrossRef
-
Wang, X. and I. Davidson, 2010. Flexible constrained spectral clustering. Proceeding of 16th ACM SIGKDD International Conference on Knowledge Discovery and Data Mining (KDD'10), pp: 563-572.
CrossRef
-
Zanghi, H., S. Volant and C. Ambroise, 2009. Clustering based on random graph model embedding vertex features. Pattern Recogn. Lett., 31(9): 830-836.
CrossRef
-
Zhou, Y., H. Cheng and J.X. Yu, 2009. Graph clustering based on structural/attribute similarities. Proceedings of the VLDB Endowment, 2(1): 718-729.
CrossRef
Competing interests
The authors have no competing interests.
Open Access Policy
This article is distributed under the terms of the Creative Commons Attribution 4.0 International License (http://creativecommons.org/licenses/by/4.0/), which permits unrestricted use, distribution, and reproduction in any medium, provided you give appropriate credit to the original author(s) and the source, provide a link to the Creative Commons license, and indicate if changes were made.
Copyright
The authors have no competing interests.
|
|
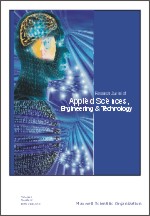 |
ISSN (Online): 2040-7467
ISSN (Print): 2040-7459 |
 |
Information |
|
|
|
Sales & Services |
|
|
|