Research Article | OPEN ACCESS
Semantic Similarity Measurement Methods: The State-of-the-art
Fatmah Nazar Mahmood and Amirah Ismail
Faculty of Information Science and Technology, Universiti Kebangsaan Malaysia, Selangor, Malaysia
Research Journal of Applied Sciences, Engineering and Technology 2014 18:1923-1932
Received: May 04, 2014 | Accepted: June 08, 2014 | Published: November 15, 2014
Abstract
With increasing importance of estimating the semantic similarity between concepts this study tries to highlight some methods used in this area. Similarity measurement between concepts has become a significant component in most intelligent knowledge management applications, especially in fields of Information Extraction (IE) and Information Retrieval (IR). Measuring similarity among concepts has been considered as a quantitative measure of the information; computation of similarity relies on the relations and the properties linked between the concepts in ontology. In this study we have briefly reviewed the main categories of semantic similarity.
Keywords:
Feature based measures, hybrid measures, information content-based measures, ontology based measures,
References
-
Agirre, E., E. Alfonseca, K. Hall, J. Kravalova, M. Pasca and A. Soroa, 2009. A study on similarity and relatedness using distributional and WordNet-based approaches. Proceeding of Human Language Technologies: The 2009 Annual Conference of the North American Chapter of the Association for Computational Linguistics. Association for Computational Linguistics, pp: 19-27.
CrossRef -
Al-Mubaid, H. and H.A. Nguyen, 2006. A cluster-based approach for semantic similarity in the biomedical domain. Proceeding of 28th Annual International Conference of the IEEE Engineering in Medicine and Biology Society (EMBS'06), pp: 2713-2717.
CrossRef -
Batet, M., D. Sánchez and A. Valls, 2011. An ontology-based measure to compute semantic similarity in biomedicine. J. Biomed. Inform., 44(1): 118-125.
CrossRef PMid:20837160 -
Bollegala, D., Y. Matsuo and M. Ishizuka, 2011. A web search engine-based approach to measure semantic similarity between words. IEEE T. Knowl. Data En., 23(7): 977-990.
CrossRef -
Budanitsky, A., 1999. Lexical semantic relatedness and its application in natural language processing. Technical Report CSRG-390, Computer Systems Research Group, University of Toronto, August.
-
Bulskov, H., 2006. Ontology-based information retrieval. Ph.D. Thesis, Roskilde University, Denmark.
-
Bulskov, H., R. Knappe and T. Andreasen, 2002. On measuring similarity for conceptual querying. In: Andreasen, T. et al. (Eds.), Flexible Query Answering Systems. LNAI 2522, Springer, Berlin, Heidelberg, pp: 100-111.
CrossRef -
Chaves-González, J.M. and J. MartíNez-Gil, 2013. Evolutionary algorithm based on different semantic similarity functions for synonym recognition in the biomedical domain. Knowl-Based Syst., 37: 62-69.
CrossRef -
Harispe, S., D. Sánchez, S. Ranwez, S. Janaqi and J. Montmain, 2013. A framework for unifying ontology-based semantic similarity measures: A study in the biomedical domain. J. Biomed. Inform., 48: 38-53.
CrossRef PMid:24269894 -
Hirst, G. and D. St-Onge, 1998. Lexical Chains as Representations of Context for the Detection and Correction of Malapropisms. In: WordNet: An Electronic Lexical Database. The MIT Press, Cambridge, pp: 305-332.
-
Hliaoutakis, A., G. Varelas, E. Voutsakis, E.G.M. Petrakis and E. Milios, 2006. Information retrieval by semantic similarity. Int. J. Semant. Web Inf., 2(3): 55-73.
CrossRef -
Jayasri, D. and D. Manimegalai, 2013. Semantic similarity measures on different ontologies: Survey and a proposal of cross ontology based similarity measure. Int. J. Sci. Res., 2(2): 455-461.
-
Jiang, J.J. and D.W. Conrath, 1997. Semantic similarity based on corpus statistics and lexical taxonomy Proceeding of International Conference Research on Computational Linguistics (ROCLING X), Taiwan.
-
Leacock, C. and M. Chodorow, 1998. Combining local context and WordNet similarity for word sense identification. WordNet: An Electronic Lexical Database, 49(2): 265-283.
-
Li, Y., Z.A. Bandar and D. McLean, 2003. An approach for measuring semantic similarity between words using multiple information sources. IEEE T. Knowl. Data En., 15(4): 871-882.
CrossRef -
Lin, D., 1998. An information-theoretic definition of similarity. Proceedings of the Fifteenth International Conference on Machine Learning (ICML, 1998), pp: 296-304.
-
McInnes, B.T. and T. Pedersen, 2013. Evaluating measures of semantic similarity and relatedness to disambiguate terms in biomedical text. J. Biomed. Inform., 46(6): 1116-1124.
CrossRef PMid:24012881 PMCid:PMC3864022 -
Nguyen, H.A., 2006. New semantic similarity techniques of concepts applied in the biomedical domain and WordNet. M.S. Thesis, University of Houston, USA.
-
Pedersen, T., S.V.S. Pakhomov, S. Patwardhan and C.G. Chute, 2007. Measures of semantic similarity and relatedness in the biomedical domain. J. Biomed. Inform., 40(3): 288-299.
CrossRef PMid:16875881 -
Pirró, G., 2009. A semantic similarity metric combining features and intrinsic information content. Data Knowl. Eng., 68(11): 1289-1308.
CrossRef -
Pirró, G. and J. Euzenat, 2010. A feature and information theoretic framework for semantic similarity and relatedness. Proceeding of the 9th International Semantic Web Conference (ISWC'10), 6496: 615-630.
CrossRef -
Quillian, M.R., 1968. Semantic Memory. In: Minsky, M. (Ed.), Semantic Information Processing. MIT Press, Cambridge.
-
Rada, R., H. Mili, E. Bicknell and M. Bletner, 1989. Development and application of a metric on semantic nets. IEEE T. Syst. Man Cyb., 19(1): 17-30.
CrossRef -
Ren, F. and D.B. Bracewell, 2009. Advanced information retrieval. Electron. Notes Theor. Comput. Sci., 225: 303-317.
CrossRef -
Resnik, P., 1995. Using information content to evaluate semantic similarity in a taxonomy Proceeding of the 14th International Joint Conference on Artificial Intelligence, pp: 448-453.
-
Sánchez, D., M. Batet, D. Isern and A. Valls, 2012. Ontology-based semantic similarity: A new feature-based approach. Expert Syst. Appl., 39(9): 7718-7728.
CrossRef -
Saruladha, K., G. Aghila and S. Raj, 2010. A survey of semantic similarity methods for ontology based information retrieval. Proceeding of 2nd International Conference on Machine Learning and Computing (ICMLC, 2010), pp: 297-301.
CrossRef -
Schickel-Zuber, V. and B. Faltings, 2007. OSS: A semantic similarity function based on hierarchical ontologies." Proceeding of the 20th International Joint Conference on Artifical Intelligence (IJCAI, 2007), 7: 551-556.
-
Scriver, A.D., 2006. Semantic distance in wordnet: A simplified and improved measure of semantic relatedness. M.A. Thesis, University of Waterloo, Canada.
-
Seco, N., T. Veale and J. Hayes, 2004. An intrinsic information content metric for semantic similarity in WordNet. Proceeding of the 16th European Conference on Artificial Intelligence (ECAI, 2004). John Wiley, Valencia, Spain.
-
Shannon, C.E., 1948. A mathematical theory of communication. Bell Syst. Tech. J., 27: 623-656.
CrossRef -
Sussna, M., 1993. Word sense disambiguation for free-text indexing using a massive semantic network. Proceeding of the 2nd International Conference on Information and Knowledge Management. ACM, New York, USA, pp: 67-74.
CrossRef -
Tversky, A., 1977. Features of similarity." Psychol. Rev., 84(4): 327. Retrieved from: http://shodhganga.inflibnet.ac.in/bitstream/10603/5300/10/10_chapter%202.pdf.
CrossRef Direct Link -
Wu, Z. and M.S. Palmer, 1994. Verb semantics and lexical selection with incomplete and imperfect judgements. Proceeding of the 15th ACM International Conference on Information and Knowledge management (CIKM'06). ACM Press, New York, pp: 102-111.
PMid:8302268
Competing interests
The authors have no competing interests.
Open Access Policy
This article is distributed under the terms of the Creative Commons Attribution 4.0 International License (http://creativecommons.org/licenses/by/4.0/), which permits unrestricted use, distribution, and reproduction in any medium, provided you give appropriate credit to the original author(s) and the source, provide a link to the Creative Commons license, and indicate if changes were made.
Copyright
The authors have no competing interests.
|
|
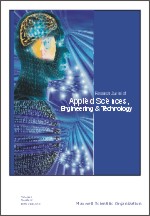 |
ISSN (Online): 2040-7467
ISSN (Print): 2040-7459 |
 |
Information |
|
|
|
Sales & Services |
|
|
|