Research Article | OPEN ACCESS
LS-SVM Based AGC of an Asynchronous Power System with Dynamic Participation from DFIG Based Wind Turbines
1Gulshan Sharma, 1K.R. Niazi and 2Ibraheem
1Department of Electrical Engineering, Malaviya National Institute of Technology, Jaipur, India
2Department of Electrical Engineering, Qassim University, Buraydah, Saudi Arabia
Research Journal of Applied Sciences, Engineering and Technology 2014 8:1022-1028
Received: May 09, 2014 | Accepted: June 16, 2014 | Published: August 25, 2014
Abstract
Modern power systems are large and interconnected with growing trends to integrate wind energy to the power system and meet the ever rising energy demand in an economical manner. The penetration of wind energy has motivated power engineers and researchers to investigate the dynamic participation of Doubly Fed Induction Generators (DFIG) based wind turbines in Automatic Generation Control (AGC) services. However, with dynamic participation of DFIG, the AGC problem becomes more complex and under these conditions classical AGC are not suitable. Therefore, a new non-linear Least Squares Support Vector Machines (LS-SVM) based regulator for solution of AGC problem is proposed in this study. The proposed AGC regulator is trained for a wide range of operating conditions and load changes using an off-line data set generated from the robust control technique. A two-area power system connected via parallel AC/DC tie-lines with DFIG based wind turbines in each area is considered to demonstrate the effectiveness of the proposed AGC regulator and compared with results obtained using Multi-Layer Perceptron (MLP) neural networks and conventional PI regulators under various operating conditions and load changes.
Keywords:
Automatic generation control , doubly fed induction generator , RBF kernel , support vector machines,
References
-
Ahamed, T.P.I., P.S.N. Rao and P.S. Sastry, 2006. Reinforcement learning controllers for automatic generation control in power systems having reheat units with GRC and dead-band. Int. J. Power Energ. Syst., 26(2): 137-46.
CrossRef -
Boolchandani, D., A. Ahmed and V. Sahula, 2011. Efficient kernel functions for support vector machine regression model for analog circuits performance evaluation. Analog Integr. Circ. S., 66: 117-128.
CrossRef -
Cavin, J.K., M.C. Budge and P. Rosmunsen, 1971. An optimal linear system approach to load frequency control. IEEE T. Power Ap. Syst., 90: 2472-2482.
CrossRef -
Demiroren, A. and E. Yesil, 2004. Automatic generation control with fuzzy logic controllers in the power system including SMES units. Int. J. Elec. Power, 26: 291-305.
CrossRef -
Keung, P., P. Lei, H. Banakar and B.T. Ooi, 2009. Kinetic energy of wind-turbine generators for system frequency support. IEEE T. Power Syst., 24(1): 279-287.
CrossRef -
Mohamed, T.H., H. Bevrani, A.A. Hassan and T. Hiyama, 2011. Decentralized model predictive based load frequency control in an interconnected power system. Energ. Convers. Manag., 52: 1208-1214.
CrossRef -
Morren, J., S.W.H. Haan, W.H. Kling and J.A. Ferreira, 2006. Wind turbines emulating inertia and supporting primary frequency control. IEEE T. Power Syst., 21(1): 433-434.
CrossRef -
Shayeghi, H. and H.A. Shayanfar, 2002. µ controller design for interconnected power systems load frequency control. Proceedings of the International Conference on PES, 352-158: 751-758.
-
Vapnik, V., 1998. Statistical Learning Theory. John Wiley, New York.
-
Wang, Y., R. Zhou and C. Wen, 1993. Robust load-frequency controller design for power system. IEE Proc-C, 140(1): 11-16.
CrossRef
Competing interests
The authors have no competing interests.
Open Access Policy
This article is distributed under the terms of the Creative Commons Attribution 4.0 International License (http://creativecommons.org/licenses/by/4.0/), which permits unrestricted use, distribution, and reproduction in any medium, provided you give appropriate credit to the original author(s) and the source, provide a link to the Creative Commons license, and indicate if changes were made.
Copyright
The authors have no competing interests.
|
|
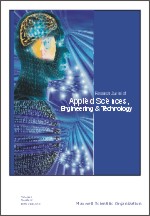 |
ISSN (Online): 2040-7467
ISSN (Print): 2040-7459 |
 |
Information |
|
|
|
Sales & Services |
|
|
|