Research Article | OPEN ACCESS
Support Vector Machine Based Pades Approximant for Diabetic Retinal Eye Detection
1S. Vijayalakshmi and 2P. Sivaprakasam
1Karpagam University, Coimbatore-641659, India
2Department of Computer Science, Sri Vasavi College, Erode 638316, India
Research Journal of Applied Sciences, Engineering and Technology 2014 20:4206-4210
Received: October 09, 2013 | Accepted: November 18, 2013 | Published: May 20, 2014
Abstract
Diabetic Retina (DR), a problem of formation of blood clot must be diagnosed at an early stage for laser therapy. A number of automated diagnosis methods based on image segmentation of fundus image is present which can diagnose DR at late mild proliferative stage. Proposed work is aimed to detect DR at early mild proliferative stage. Method uses feature extraction of fundus image using 2D Gabor filtering and pre-classification for feature vector extraction using Pades approximation. The Padesvector are then again classified using SVM by forming a dual of convex quadratic type minimization problem for linearly separable hyper plane. The performance of the proposed work is tested with set of images taken from fundus camera.
Keywords:
Diabetic Retina (DR), lagrangians multiplier, pades approximation, Support Vector Machines (SVM),
References
-
Alauddin, B., N. Baikunth, C. Joselito and K. Ramamohanarao, 2007. Blood vessel segmentation from color retinal images using unsupervised texture classification. IEEE Image Proc., 5(1): 521-524.
-
Alireza, O., M. Majid, T. Barry and M. Richard, 2002. Comparative exudate classification using support vector machines and neural networks. Lect. Note Comput. Sc., 2489: 413-420.
CrossRef
-
Berrichi, F.Z. and M. Benyettou, 2009. Automated diagnosis of retinal images using the Support Vector Machine (SVM). A White Paper. Laboratory Modeling and Optimization of Systemes Industriels: Lamosi. Department of Informatics, Faculty of Science, USTO. BP 1505 EL M'naouer 31000 Oran, Algerie, pp: 1-6.
-
Carmen, A.L.C. and T. Domenico, 2012. Graph-based minimal path tracking in the skeleton of the retinal vascular network. Proceeding of the 2012 25th International Symposium on Computer-based Medical Systems (CBMS). Rome, 1: 1-6.
-
Diego, M., A. Arturo, E. Manuel, A. Gegúndez and M.B. José, 2011. A new supervised method for blood vessel segmentation in retinal images by using gray-level and moment invariants-based features. IEEE T. Med. Imaging, 30(1): 146-158.
CrossRef PMid:20699207
-
Ding, L., Y. Zhang and X. Zhang, 2011. An approach to retinal image segmentations using fuzzy clustering in combination with morphological filters. Proceeding of the 30th Chinese Control Conference, pp: 3062-3065.
-
Giri, B.K., S.T. Satya and P.V. Subbaiah, 2008. Retinal vessel segmentation using spatially weighted fuzzy c-means clustering and histogram matching. Proceeding of the Annual IEEE India Conference (INDICON 2008). Kanpur, 1: 1-6.
-
Jian, M., L. Xiaomin, M.K. Malgorzata, X. Zhiliang, S.A. Mark, D.R. Elliot and Z.C. Danny, 2009. Segmentation, reconstruction and analysis of blood thrombus formation in 3D 2-photon microscopy images. Proceeding of the 22nd IEEE International Symposium on Computer-based Medical Systems, 1: 1-8.
-
Mona, K.G., D. Michael, Abràmoff, K. Randy, R.R. Stephen, W. Xiaodong and S. Milan, 2008. Intraretinal layer segmentation of macular optical coherence tomography images using optimal 3-D graph search. IEEE T. Med. Imaging, 27(10): 1495-1505.
-
Muhammad, M.F., R. Paolo, H. Andreas, U. Bunyarit, R.R. Alicja, G.O. Christopher and A.B. Sarah, 2012. An ensemble classification-based approach applied to retinal blood vessel segmentation. IEEE T. Biomed. Eng., 59(9): 2538-2548.
CrossRef
-
Peng, B., L. Zhang and Z. David, 2011. Automatic image segmentation by dynamic region merging. IEEE Image Proc., 20(12): 3592-3605.
CrossRef PMid:21609885
-
Selvathi, D. and V. Lalitha, 2011. Gabor wavelet based blood vessel segmentation in retinal images using kernel classifiers. Proceeding of the IEEE International Conference on Signal Processing, Communication, Computing and Networking Technologies (ICSCCN), 1: 830-835.
CrossRef
-
Sharon, E., A. Brandt and R. Basri, 2000. Fast multiscale image segmentation. Proceeding of the IEEE Conference on Computer Vision and Pattern Recognition, 1: 70-77.
CrossRef
-
Shi, J. and J. Malik, 2000. Normalized cuts and image segmentation. IEEE T. Pattern Anal., 22(8): 888-905.
CrossRef
-
Simon, B.E., M. Victor, A. Carla, P. Marios, B. Wendall, Z. Gilberto and S. Peter, 2010. Automatic system for diabetic retinopathy screening based on AM-FM, partial least squares and support vector machines. Proceeding of the 2010 IEEE International Symposium on Biomedical Imaging: From Nano to Macro. Rotterdam, 1: 1349-1352.
Competing interests
The authors have no competing interests.
Open Access Policy
This article is distributed under the terms of the Creative Commons Attribution 4.0 International License (http://creativecommons.org/licenses/by/4.0/), which permits unrestricted use, distribution, and reproduction in any medium, provided you give appropriate credit to the original author(s) and the source, provide a link to the Creative Commons license, and indicate if changes were made.
Copyright
The authors have no competing interests.
|
|
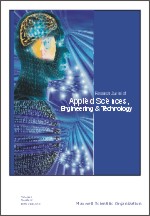 |
ISSN (Online): 2040-7467
ISSN (Print): 2040-7459 |
 |
Information |
|
|
|
Sales & Services |
|
|
|