Research Article | OPEN ACCESS
A Statistical Approach of Texton Based Texture Classification Using LPboosting Classifier
1C. Vivek and 2S. Audithan
1PRIST University, Tanjore, Tamilnadu, India
2Department of Computer Science and Engineering, PRIST University, Tamilnadu, India
Research Journal of Applied Sciences, Engineering and Technology 2014 19:4088-4094
Received: December 04, 2013 | Accepted: January 01, 2014 | Published: May 15, 2014
Abstract
The aim of the study in this research deals with the accurate texture classification and the image texture analysis has a voluminous errand prospective in real world applications. In this study, the texton co-occurrence matrix applied to the Broadatz database images that derive the template texton grid images and it undergoes to the discrete shearlet transform to decompose the image. The entropy lineage parameters of redundant and interpolate at a certain point which congregating adjacent regions based on geometric properties then the classification is apprehended by comparing the similarity between the estimated distributions of all detail sub bands through the strong LP boosting classification with various weak classifier configurations. We show that the resulted texture features while incurring the maximum of the discriminative information. Our hybrid classification method significantly outperforms the existing texture descriptors and stipulates classification accuracy in the state-of-the-art real world imaging applications.
Keywords:
LPboost classifier, shearlet transform, texton co-occurrence matrix, texture image classification, weak classification,
References
-
Chen, C.H. and G.K. Young, 1982. A study of texture classification using spectral features. Technical Report F SMU-EE-TR-82-1.
-
Easley, G., D. Labate and W.Q. Lim, 2008. Sparse directional image representations using the discrete shearlet transform. Appl. Comput. Harmon. A., 25: 25-46.
CrossRef
-
Fang, Y., Y. Fu, C. Sun and J. Zhou, 2011. Improved boosting algorithm using combined weak classifiers. J. Comput. Inform. Syst., 7(5): 1455-1462.
-
Fang, Y.K., Y. Fu, C.J. Sun and J.L. Zhou, 2010. LPBoost with strong classifiers. Int. J. Comput. Intell. Syst., 3(Sup01): 88-100.
-
Haralick, R.M., K. Shanmugam and I.H. Dinstein, 1973. Textural features for image classification. IEEE T. Syst. Man Cyb., 6: 610-621.
CrossRef
-
Hinrichs, C., V. Singh, L. Mukherjee, G. Xu, M.K. Chung and S.C. Johnson, 2009. Spatially augmented LPboosting for AD classification with evaluations on the ADNI dataset. Neuroimage, 48(1): 138-149.
CrossRef PMid:19481161 PMCid:PMC2773131
-
Julesz, B., 1981. Textons, the elements of texture perception and their interactions. Nature, 290: 91-97.
CrossRef PMid:7207603
-
Liu, G.H., Z.Y. Li, L. Zhang and Y. Xu, 2011. Image retrieval based on micro-structure descriptor. Pattern Recogn., 44(9): 2123-2133.
CrossRef
-
Liu, G.H., L. Zhang, Y.K. Hou, Z.Y. Li and J.Y. Yang, 2010. Image retrieval based on multi-texton histogram. Pattern Recogn., 43(7): 2380-2389.
CrossRef
-
Monay, F. and D. Gatica-Perez, 2007. Modeling semantic aspects for cross-media image indexing. IEEE T. Pattern Anal., 29(10): 1802-1817.
CrossRef PMid:17699924
-
Randen, T. and J.H. Husoy, 1999. Filtering for texture classification: A comparative study. IEEE T. Pattern Anal., 21(4): 291-310.
CrossRef
-
Smith, J.R. and S.F. Chang, 1994. Transform features for texture classification and discrimination in large image databases. Proceedings of the IEEE International Conference on Image Processing (ICIP-94), 3: 407-411.
CrossRef
-
Tou, J.Y., Y.H. Tay and P.Y. Lau, 2009. Recent trends in texture classification: A review. Proceedings of the Symposium on Progress in Information and Communication Technology. Kuala Lumpur, Malaysia, December 7-8.
-
Unser, M., 1986. Local linear transforms for texture measurements. Signal Process., 11(1): 61-79.
CrossRef
-
Unser, M., 1995. Texture classification and segmentation using wavelet frames. IEEE T. Image Process., 4(11): 1549-1560.
CrossRef PMid:18291987
-
Van de Wouwer, G., 1998. Wavelets for multiscale texture analysis. Ph.D. Thesis, University of Antwerp, pp: 1-162.
-
Vivek, C. and S. Audithan, 2014. Texture classification by shearlet Band Signatures. Asian J. Sci. Res. Retrieved from: scialert.net/abstract/?doi=ajsr. 0000.51616.51616?.
-
Wang, Z.Z. and J.H. Yong, 2008. Texture analysis and classification with linear regression model based on wavelet transform. IEEE T. Image Process., 17(8): 1421-1430.
CrossRef PMid:18632350
Competing interests
The authors have no competing interests.
Open Access Policy
This article is distributed under the terms of the Creative Commons Attribution 4.0 International License (http://creativecommons.org/licenses/by/4.0/), which permits unrestricted use, distribution, and reproduction in any medium, provided you give appropriate credit to the original author(s) and the source, provide a link to the Creative Commons license, and indicate if changes were made.
Copyright
The authors have no competing interests.
|
|
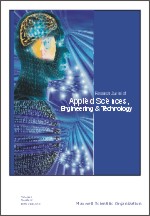 |
ISSN (Online): 2040-7467
ISSN (Print): 2040-7459 |
 |
Information |
|
|
|
Sales & Services |
|
|
|