Research Article | OPEN ACCESS
Neural Gen Feature Selection for Supervised Learning Classifier
1Mohammed Hasan Abdulameer, 1Siti Norul Huda Sheikh Abdullah and 2Zulaiha Ali Othman
1Pattern Recognition Research Group, Centre for Artificial Intelligence Technology, Faculty of Information Science and Technology
2Data Mining and Optimization Group, Faculty of Information System and Technology, Universiti Kebangsaan Malaysia, 43600 Bandar Baru Bangi, Malaysia
Research Journal of Applied Sciences, Engineering and Technology 2014 15:3181-3187
Received: November 04, 2013 | Accepted: November 26, 2013 | Published: April 19, 2014
Abstract
Face recognition has recently received significant attention, especially during the past few years. Many face recognition techniques were developed such as PSO-SVM and LDA-SVM However, inefficient features in the face recognition may lead to inadequate in the recognition results. Hence, a new face recognition system based on Genetic Algorithm and FFBNN technique is proposed. Our proposed face recognition system initially performs the feature extraction and these optimal features are promoted to the recognition process. In the feature extraction, the optimal features are extracted from the face image database by Genetic Algorithm (GA) with FFBNN and the computed optimal features are given to the FFBNN technique to carry out the training and testing process. The optimal features from the feature database are fed to the FFBNN for accomplishing the training process. The well trained FFBNN with the optimal features provide the recognition result. The optimal features in FFBNN by GA efficiently perform the face recognition process. The human face dataset called YALE is utilized to analyze the performance of our proposed GA-FFNN technique and also this GA-FFBNN is compared with standard SVM and PSO-SVM techniques.
Keywords:
Face recognition, FFBNN, GA, PCA, SVM,
References
-
Anil, K.J. and A. Kumar, 2010. Biometrics of Next Generation: An Overview. Second Generation Biometrics, Springer, Heidelberg.
-
Anna, D. and A. Saro, 2008. Image compression with back-propagation neural network using cumulative distribution function. World Acad. Sci. Eng. Technol., 17: 1075-1079.
-
Balcoh, N.K., M.H. Yousaf, W. Ahmad and I. Baig, 2012. Algorithm for efficient attendance management: Face recognition based approach. Int. J. Comput. Sci., 9(4).
-
Belghini, N., A. Zarghili, J. Kharroubi and A. Majda, 2012. Learning a back propagation neural network with error function based on bhattacharyya distance for face recognition. Int. J. Image Graph. Signal Process., 4(8): 8-14.
CrossRef
-
Ephzibah, E.P., 2011. Cost effective approach on feature selection using genetic algorithms and fuzzy logic for diabetes diagnosis. Int. J. Soft Comput., 2(1): 1-10.
CrossRef
-
Gordon, G.G., 1992. Face recognition based on depth and curvature features. Proceedings of IEEE Computer Society Conference on Computer Vision and Pattern Recognition. Champaign, IL, USA, pp: 808-810.
CrossRef
-
Guillaumin, M., T. Mensink, J. Verbeek and C. Schmid, 2011. Face recognition from caption-based supervision. Int. J. Comput. Vision, 96(1): 64-82.
CrossRef
-
Hirdesh, K. and Padmavati, 2012. Face recognition using SIFT by varying distance calculation matching method. Int. J. Comput. Appl., 47(3): 20-26.
-
Holland, J.H., 1992. Adaptation in Natural and Artificial Systems. MIT Press, Cambridge, MA.
-
Hussein, R., 2011. Face recognition using principle component analysis with different distance classifiers. Int. J. Comput. Sci. Network Secur., 11(10): 134-144.
-
Jennifer, H., B. Heisele and V. Blanz, 2003. Component-based face recognition with 3D morphable models. Proceedings of the 4th International Conference on Audio-and Video-Based Biometric Person Authentication (AVBPA, 2003). Guildford, UK, pp: 27-34.
-
Jin, W., J. Zhang and X. Zhang, 2011. Face recognition method based on support vector machine and particle swarm optimization. Expert Syst. Appl., 38: 4390-4393.
CrossRef
-
Kekre, H.B., S. Thepade, S. Khandelwal, A. Azmi and K. Dhamejani, 2012. Implementation of multilevel block truncation based face recognition on intermediate-4 and intermediate-9 levels. Int. J. Comput. Appl., 50(20): 39-44.
-
Khan, N.M., R. Ksantini, I.S. Ahmad and B. Boufama, 2012. A novel SVM+NDA model for classification with an application to face recognition. Pattern Recogn., 45(1): 66-79.
CrossRef
-
Li, W., L. Liu and W. Gong, 2011. Multi-objective uniform design as a SVM model selection tool for face recognition. Expert Syst. Appl., 38(6): 6689-6695.
CrossRef
-
Mini, S.A. and S. Chhabra, 2011. Effect of distance measures in PCA based face recognition. Int. J. Enterprise Comput. Bus. Syst., 1(2).
-
Patil, A.M., S.R. Kolhe and P.M. Patil, 2010. 2D face recognition techniques: A survey. Int. J. Mach. Intell., 2(1): 74-83.
-
Reza, E., A. Jahani, A. Amiri and M. Nazari, 2011. Expression-independent face recognition using biologically inspired features. Indian J. Comput. Sci. Eng., 2(3): 492-499.
-
Sanghoon, K., S.T. Chung, S. Jung, S. Jeon, J. Kim and S. Cho, 2007. Robust face recognition using AAM and Gabor features. World Acad. Sci. Eng. Technol., 33: 117-121.
-
Sudhir, K. and S. Banerji, 2011. Face recognition using K2DSPCA. Proceeding of the International Conference on Information and Network Technology. Singapore, pp: 84-88.
-
Vaseghi, S. and H. Jetelova, 2006. Principal and independent component analysis in image processing. Proceeding of the 14th ACM International Conference on Mobile Computing and Networking. San Francisco, Calif, USA, pp: 1-5.
PMid:16399044
Competing interests
The authors have no competing interests.
Open Access Policy
This article is distributed under the terms of the Creative Commons Attribution 4.0 International License (http://creativecommons.org/licenses/by/4.0/), which permits unrestricted use, distribution, and reproduction in any medium, provided you give appropriate credit to the original author(s) and the source, provide a link to the Creative Commons license, and indicate if changes were made.
Copyright
The authors have no competing interests.
|
|
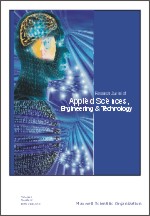 |
ISSN (Online): 2040-7467
ISSN (Print): 2040-7459 |
 |
Information |
|
|
|
Sales & Services |
|
|
|