Research Article | OPEN ACCESS
Automatic Isolated-Word Arabic Sign Language Recognition System Based on Time Delay Neural Networks
1Feras Fares Al Mashagba, 2Eman Fares Al Mashagba and 1Mohammad Othman Nassar
1Amman Arab University, Amman, Jordan
2Zarqa Private University, Zarqa, Jordan
Research Journal of Applied Sciences, Engineering and Technology 2014 11:2261-2265
Received: June 17, 2013 | Accepted: July 12, 2013 | Published: March 20, 2014
Abstract
There have been a little number of attempts to develop an Arabic sign recognition system that can be used as a communication means between hearing-impaired and other people. This study introduces the first automatic isolated-word Arabic Sign Language (ArSL) recognition system based on Time Delay Neural Networks (TDNN). The proposed vision-based recognition system that the user wears two simple but different colors gloves when performing the signs in the data sets within this study. The two colored regions are recognized and highlighted within each frame in the video to help in recognizing the signs. This research uses the multivariate Gaussian Mixture Model (GMM) based on the characteristics of the well known Hue Saturation Lightness Model (HIS) in determining the colors within the video frames. In this research the mean and covariance of the three colored region within the frames are determined and used to help us in segmenting each frame (picture) into two colored regions and outlier region. Finally we propose, create and use the following four features as an input to the TDNN; the centroid position for each hand using the center of the upper area for each frame as references, the change in horizontal velocity of both hands across the frames, the change in vertical velocity of both hands across the frames and the area change for each hand across the frames. A large set of samples has been used to recognize 40 isolated words coded by 10 different signers from the Standard Arabic sign language signs. Our proposed system obtains a word recognition rate of 70.0% in testing set.
Keywords:
Arabic signs, isolated-word based system, Jordan accent, sign language recognition systems, TDNN, video based system,
References
-
AL-Rousan, M., K. Assaleh and A. Tala'a, 2009. Videobased signer-independent Arabic sign language recognition using hidden Markov models. J. Appl. Soft Comput., 9(3): 990-999.
CrossRef
-
AL-Rousan, M., O. Al-Jarrah and N. Nayef, 2007. Neural networks based recognition system for isolated arabic sign language. Proceeding of International Conference on Information Technology (ICIT, 2007), pp: 345.
-
Charayaphan, C. and A. Marble, 1992. Image processing system for interpreting motion in American Sign Language. J. Biomed. Eng., 14: 419-425.
CrossRef
-
Khaled, A., S. Tamer and Z. Mohammed, 2012. Low complexity classification system for glove-based Arabic sign language recognition. Lect. Notes Comput. Sc, 7665: 262-268.
CrossRef
-
Kramer, J. and L. Leifer, 1988. The talking glove: An expressive and receptive "Verbal" communication aid for the deaf, deaf-blind and Nonvocal. SIGCAPH, 39(Spring): 12-15.
CrossRef
-
Manar, M., A.Z. Farid, D. Mufleh and A.Z. Raed, 2012. Recognition of Arabic Sign Language (ArSL) using recurrent neural networks. J. Intell. Learn. Syst. Appl., 4: 41-52.
-
Mohandes, M. and M. Deriche, 2005. Image based Arabic sign language recognition. Proceedings of the 8th International Symposium on Signal Processing and its Applications, pp: 86- 89.
CrossRef
-
Nadia, R.A. and M.A. Yasser, 2012. Real-Time Arabic Sign Language (ArSL) recognition. Proceeding of the International Conference on Communications and Information Technology (ICCIT-2012), Tunisia, pp: 497-501.
-
Reyadh, N., H.O. Hussein and J. Shaimaa, 2012. Development of a new Arabic Sign Language recognition using K-nearest neighbor algorithm. J. Emerg. Trends Comput. Inform. Sci., 3(8).
-
Samir, A. and M. Abul-Ela, 2012. 3D Arabic sign language recognition using linear combination of multiple 2D views. Proceeding of the 8th International Conference on Informatics and Systems (INFOS), pp: 6-13.
-
Sandjaja, I. and N. Marcos, 2009. Sign language number recognition. Proceeding of the 5th International Joint Conference on INC, IMS and IDC, pp: 1503-1508.
CrossRef
-
Shanableh, T., K. Assaleh and M. Al-Rousan, 2007. Spatio-temporal feature extraction techniques for isolated gesture recognition in Arabic sign language. IEEE T. Syst. Man Cy. B, 37(3): 641-650.
CrossRef
-
Tolba, M.F., S. Ahmed and A.E. Magdy, 2012. Arabic sign language continuous sentences recognition using PCNN and graph matching. Neural Comput. Appl. J., 2012: 1-12.
Competing interests
The authors have no competing interests.
Open Access Policy
This article is distributed under the terms of the Creative Commons Attribution 4.0 International License (http://creativecommons.org/licenses/by/4.0/), which permits unrestricted use, distribution, and reproduction in any medium, provided you give appropriate credit to the original author(s) and the source, provide a link to the Creative Commons license, and indicate if changes were made.
Copyright
The authors have no competing interests.
|
|
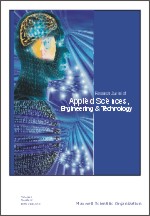 |
ISSN (Online): 2040-7467
ISSN (Print): 2040-7459 |
 |
Information |
|
|
|
Sales & Services |
|
|
|