Research Article | OPEN ACCESS
AR-based Algorithms for Short Term Load Forecast
Zuhairi Baharudin, Mohd. Azman Zakariya, Mohd. HarisMdKhir, Perumal Nallagownden and Muhammad Qamar Raza
Electrical and Electronics Department, Universiti Teknologi PETRONAS, 31750 Tronoh, Perak, Malaysia
Research Journal of Applied Sciences, Engineering and Technology 2014 6:1223-1229
Received: March 28, 2013 | Accepted: April 15, 2013 | Published: February 15, 2014
Abstract
Short-term load forecast plays an important role in planning and operation of power systems. The accuracy of the forecast value is necessary for economically efficient operation and effective control of the plant. This study describes the methods of Autoregressive (AR) Burg’s and Modified Covariance (MCOV) in solving the short term load forecast. Both algorithms are tested with power load data from Malaysian grid and New South Wales, Australia. The forecast accuracy is assessed in terms of their errors. For the comparison the algorithms are tested and benchmark with the previous successful proposed methods.
Keywords:
Artificial neural network, Autoregressive (AR), linear predictor, Short Term Load Forecast (STLF),
References
-
Amarawickrama, H.A. and L.C. Hunt, 2008. Electricity demand for Sri Lanka: A time series analysis. Energy, 33(5): 724-739.
CrossRef
-
Baharudin, Z. and N.S. Kamel, 2007. One week ahead short term load forecasting. Proceeding of the 7th IASTED European Conference on Power and Energy Systems (EuroPES 2007). Palma de Mallorca, Spain, pp: 582-117.
-
Baharudin, Z. and N.S. Kamel, 2008. A.R. modified covariance in one week ahead short term load forecast. Proceeding of the 2nd IASTED Asian Conference on Power and Energy Systems. Langkawi, Malaysia, pp: 606-070.
-
Chakhchoukh, Y., P. Panciatici and L. Mili, 2011. Electric load forecasting based on statistical robust methods. IEEE T. Power Syst., 26(3): 982-991.
CrossRef
-
Darbellay, G.A. and M. Slama, 2000. Forecasting the short-term demand for electricity: Do neural networks stand a better chance? Int. J. Forecasting, 16(1): 71-83.
CrossRef
-
De Felice, M. and Y. Xin, 2011. Short-term load forecasting with neural network ensembles: A comparative study [Application Notes]. IEEE Comput. Intell. M., 6(3): 47-56.
CrossRef
-
De la Torre, S., A.J. Conejo and J. Contreras, 2008. Transmission expansion planning in electricity markets. IEEE T. Power Syst., 23(1): 238-248.
CrossRef
-
Del-Carpio Huayllas, T.E. and D.S. Ramos, 2010. Electric power forecasting methodologies of some south American countries: A comparative analysis. IEEE (Revista IEEE America Latina) Latin Am. T., 8(5): 519-525./>
CrossRef
-
El-Telbany, M. and F. El-Karmi, 2008. Short-term forecasting of Jordanian electricity demand using particle swarm optimization. Electr. Pow. Syst. Res., 78(3): 425-433.
CrossRef
-
Hanmandlu, M. and B.K. Chauhan, 2011. Load forecasting using hybrid models. IEEE T. Power Syst., 26(1): 20-29.
CrossRef
-
Hyndman, R.J. and F. Shu, 2010. Density forecasting for long-term peak electricity demand. IEEE T. Power Syst., 25(2): 1142-1153.
CrossRef
-
Lira, F., C. Munoz, F. Nunez and A. Cipriano, 2009. Short-term forecasting of electricity prices in the colombian electricity market. IET Gener. Transm. Dis., 3(11): 980-986.
CrossRef
-
Maksimovich, S.M. and V.M. Shiljkut, 2009. The peak load forecasting afterwards its intensive reduction. IEEE T. Power Deliver, 24(3): 1552-1559.
CrossRef
-
Qun, Z., L. Tesfatsion and L. Chen-Ching, 2011. Short-term congestion forecasting in wholesale power markets. IEEE T. Power Syst., 26(4): 2185-2196.
CrossRef
Competing interests
The authors have no competing interests.
Open Access Policy
This article is distributed under the terms of the Creative Commons Attribution 4.0 International License (http://creativecommons.org/licenses/by/4.0/), which permits unrestricted use, distribution, and reproduction in any medium, provided you give appropriate credit to the original author(s) and the source, provide a link to the Creative Commons license, and indicate if changes were made.
Copyright
The authors have no competing interests.
|
|
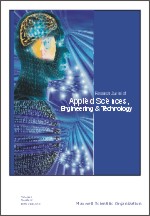 |
ISSN (Online): 2040-7467
ISSN (Print): 2040-7459 |
 |
Information |
|
|
|
Sales & Services |
|
|
|