Research Article | OPEN ACCESS
A Wavelet Neural Network Hybrid Model for Monthly Ammonia Forecasting in River Water
1Yi Wang, 1Yuanyuan Wang, 1, 2Liang Guo, 1, 2Ying Zhao, 1Zhichao Zhang and 1, 2Peng Wang
1School of Municipal and Environment Engineering, Harbin Institute
of Technology, Harbin, 150090, Heilongjiang Province, China
2State Key Laboratory of Urban Water Resource and Environment, Harbin Institute of Technology, Harbin 150090, China
Research Journal of Applied Sciences, Engineering and Technology 2013 2:345-348
Received: November 20, 2012 | Accepted: December 15, 2012 | Published: June 10, 2013
Abstract
Forecasting water quality is always an effective approach for water environmental management. This study presents a combined Wavelet transform (WA) and Artificial Neural Network (ANN) model for monthly ammonia nitrogen series prediction in river water. The WA decomposed original time series into different subseries, in which the most significant one was chosen as the training data instead of the original series. Compared to the traditional ANN, the WA-ANN models were found more accurate and reliable. The results of the study indicate that WA could remove the noise of the original datasets and the WA-ANN could help environment decision-maker manage water quality more effective.
Keywords:
Artificial neural network, environment management, Harbin region, time series, water quality forecasting, wavelet analysis,
Competing interests
The authors have no competing interests.
Open Access Policy
This article is distributed under the terms of the Creative Commons Attribution 4.0 International License (http://creativecommons.org/licenses/by/4.0/), which permits unrestricted use, distribution, and reproduction in any medium, provided you give appropriate credit to the original author(s) and the source, provide a link to the Creative Commons license, and indicate if changes were made.
Copyright
The authors have no competing interests.
|
|
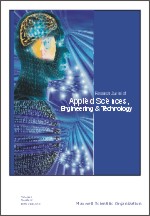 |
ISSN (Online): 2040-7467
ISSN (Print): 2040-7459 |
 |
Information |
|
|
|
Sales & Services |
|
|
|