Research Article | OPEN ACCESS
Improving Efficiency of Classification using PCA and Apriori based Attribute Selection Technique
1K. Rajeswari, 2Rohit Garud and 3V. Vaithiyanathan
1Pimpri Chinchwad College of Engineering, Pune and Ph. D Research Scholar, SASTRA University, Tanjore, India
2Pimpri Chinchwad College of Engineering, Pune, India
3Professor and Associate Dean Research, SASTRA University, Tanjore, India
Research Journal of Applied Sciences, Engineering and Technology 2013 24:4681-4684
Received: April 09, 2013 | Accepted: May 03, 2013 | Published: December 25, 2013
Abstract
The aim of this study is to select significant features that contribute for accuracy in classification. Data mining is a field where we find lots of data which can be useful or useless in any form available in Data Warehouse. Implementing classification on these huge, uneven, useless data sets with large number of features is just a waste of time degrading the efficiency of classification algorithms and hence the results are not much accurate. Hence we propose a system in which we first use PCA (Principal Component Analysis) for selection of the attributes on which we perform Classification using Bayes theorem, Multi-Layer Perceptron, Decision tree J48 which indeed has given us better result than that of performing Classification on the huge complete data sets with all the attributes. Also association rule mining using traditional Apriori algorithm is experimented to find out sub set of features related to class label. The experiments are conducted using WEKA 3.6.0 Tool.
Keywords:
Apriori, bayes, classification, data mining, decision tree classifier j48, features, mult layer perceptron, WEKA 3.6.0,
Competing interests
The authors have no competing interests.
Open Access Policy
This article is distributed under the terms of the Creative Commons Attribution 4.0 International License (http://creativecommons.org/licenses/by/4.0/), which permits unrestricted use, distribution, and reproduction in any medium, provided you give appropriate credit to the original author(s) and the source, provide a link to the Creative Commons license, and indicate if changes were made.
Copyright
The authors have no competing interests.
|
|
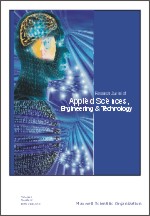 |
ISSN (Online): 2040-7467
ISSN (Print): 2040-7459 |
 |
Information |
|
|
|
Sales & Services |
|
|
|