Research Article | OPEN ACCESS
Classification Based on Attribute Positive Correlation and Average Similarity of Nearest Neighbors
Zhongmei Zhou, Guiying Pan and Xuejun Wang
Department of Computer Science and Engineering, Zhangzhou Normal University, Zhangzhou, 363000, China
Research Journal of Applied Sciences, Engineering and Technology 2013 4:1420-1423
Received: July 12, 2012 | Accepted: August 15, 2012 | Published: February 01, 2013
Abstract
The K-Nearest Neighbor algorithm (KNN) is a method for classifying objects based on the k closest training objects. An object is classified by a majority vote of its nearest neighbors. “Closeness” is defined in terms of the similarity measure between two objects. KNN is not only simple, but also sometimes has high accuracy. However, the quality of KNN classification result depends on the similarity measure between two objects and the selection of k. Moreover, the average similarity of the majority nearest neighbors may be less than the one of the minority nearest neighbors. To deal with these problems, in this study, we propose a new classification approach called APCAS: classification based on the attribute values which are positively correlated with one of the class labels and the average similarity of the nearest neighbors in each class. First, we define a new similarity measure based on the attribute values which are positively correlated with one of the class labels. Second, we classify a new object using the average similarity of the nearest neighbors in each class without selecting k. Experimental results on the mushroom data show that APCAS achieves high accuracy.
Keywords:
KNN, nearest neighbor, positive correlation, similarity measure,
Competing interests
The authors have no competing interests.
Open Access Policy
This article is distributed under the terms of the Creative Commons Attribution 4.0 International License (http://creativecommons.org/licenses/by/4.0/), which permits unrestricted use, distribution, and reproduction in any medium, provided you give appropriate credit to the original author(s) and the source, provide a link to the Creative Commons license, and indicate if changes were made.
Copyright
The authors have no competing interests.
|
|
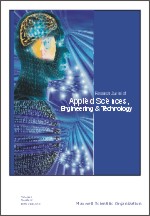 |
ISSN (Online): 2040-7467
ISSN (Print): 2040-7459 |
 |
Information |
|
|
|
Sales & Services |
|
|
|