Research Article | OPEN ACCESS
Mining and Exploring the Structure of Academic Tweets
Norah Farooqi, AlaaAlmelibari and TahaniAlsubait
Umm Al-Qura University, Makkah, Saudi Arabia
Research Journal of Applied Sciences, Engineering and Technology 2018 4:164-173
Received: January 3, 2018 | Accepted: March 2, 2018 | Published: April 15, 2018
Abstract
This paper aims to study the structure of tweets in academic accounts to ascertain the most effective form(s) of tweets. It determines the attributes which are usually used and evaluates the impacts on tweets' interactivities. Since the use of the micro blogging platform Twitter has spread globally and developed rapidly in the last few years. Users engage it in all aspects of their lives including education, economy and healthcare. Thus understanding data analytics in Twitter improves related environments, providing fruitful feedback, developing procedures and enhancing information management in organizations. Mining data types in tweets and following their feedback leads to clarifying the importance of each attribute. The reported findings present the recommended structure of tweets in academic environments due to their impact on users. This research offers a helpful guide to develop scholarly Twitter accounts and to support them to be more interactive.
Keywords:
Academic accounts, mining attributes, social media, twitter, twitter analytics,
References
-
Agrawal, R., S. Gollapudi, A. Kannan and K. Kenthapadi, 2012. Data mining for improving textbooks. ACM SIGKDD Explorations Newsletter, 13(02): 7-19.
CrossRef -
Bhulai, S., P. Kampstra, L. Kooiman, G. Koole, M. Deurloo and B. Kok, 2012. Trend visualization on Twitter: What's hot and what's not? Proceeding of the1st International Conference on Data Analytics, pp: 43-48.
Direct Link -
Carpenter, D.M., J.W. Robertson, M.E. Johnson and S. Blum, 2014. Social media analytics in education: What is it, how is it useful, and what does it tell us about how schools are discussed in social media? J. School Pub. Relat., 35: 7-43.
Direct Link -
Cummings, R.G. and M. Hsu, 2007. The effects of student response systems on performance and satisfaction: An investigation in a tax accounting class. J. College Teach. Learn., 4(12): 21-26.
Direct Link -
Denker, K.J., 2013. Student response systems and facilitating the large lecture basic communication course: Assessing engagement and learning. J. Commun. Teach., 27(1): 50-69.
CrossRef -
Kang, U., D.H. Chau and C. Faloutsos, 2011. Mining large graphs: Algorithms, inference, and discoveries. Proceeding of the 27th International Conference on Data Engineering (ICDE'11), pp: 243-254.
CrossRef -
Leong, C.K., Y.H. Lee and W.K. Mak, 2012. Mining sentiments in SMS texts for teaching evaluation. Exp. Syst. Appl., 39(3): 2584-2589.
CrossRef -
Mathioudakis, M. and N. Koudas, 2010. Twitter monitor: Trend detection over the twitter stream. Proceeding of the 2010 ACM SIGMOD International Conference on Management of Data (ACM), pp: 1155-1158.
CrossRef -
Plachouras, V. and Y. Stavrakas, 2012. Querying term associations and their temporal evolution in social data. Proceeding of the 1st International VLDB Workshop on Online Social Systems (WOSS 2012), Istanbul.
PMCid:PMC3421626 -
Porterfield, K. and M. Carnes, 2012. School communication in the age of Google. J. School Pub. Relat., 33(2): 115-130.
-
Smith, M. and V. Barash, 2008. Social SQL: Tools for exploring social databases. IEEE Data Eng., 31: 50-57.
-
Taboada, M., J. Brooke, M. Tofiloski, K. Voll and M. Stede, 2011. Lexicon-based methods for sentiment analysis. Comput. Linguist., 37(2): 267-307.
CrossRef -
Vohra, S. and J. Teraiya, 2013. Applications and challenges for sentiment analysis: A survey. Int. J. Eng. Res. Technol., 2(2): 1-5.
Direct Link -
Wang, Y., 2013. Social media in schools: a treasure trove or hot potato? J. Cases Edu. Leadership, 16(1): 83-91.
CrossRef -
Zeng, D., H. Chen, R. Lusch and S.H. Li, 2014. Social media analytics and intelligence. IEEE Intell. Syst., 25(6): 13-16.
CrossRef
Competing interests
The authors have no competing interests.
Open Access Policy
This article is distributed under the terms of the Creative Commons Attribution 4.0 International License (http://creativecommons.org/licenses/by/4.0/), which permits unrestricted use, distribution, and reproduction in any medium, provided you give appropriate credit to the original author(s) and the source, provide a link to the Creative Commons license, and indicate if changes were made.
Copyright
The authors have no competing interests.
|
|
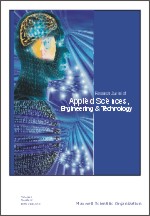 |
ISSN (Online): 2040-7467
ISSN (Print): 2040-7459 |
 |
Information |
|
|
|
Sales & Services |
|
|
|