Research Article | OPEN ACCESS
Modeling and Simulation of Double Gate Field Plate In0.2Ga0.8As/Al0.3Ga0.7 as HEMT using Gaussian Process Regression for Sensor Application`
Yousfi Abderrahim, Dibi Zohir, Guermoui Mawloud and Aissi Salim
Department of Electronics, Advanced Electronic Laboratory (LEA), Batna-2-University, Avenue Mohamed El-Hadi Boukhlouf, 05000, Batna, Algeria
Research Journal of Applied Sciences, Engineering and Technology 2017 3:112-118
Received: November 21, 2016 | Accepted: February 13, 2017 | Published: March 15, 2017
Abstract
We propose a new approach for modeling a High Electron Mobility Transistor (HEMT) using that of Gaussian Process Regression one (GPR), to improve the current-voltage characteristics of HEMT transistor for using in electronic and biological domain or any other domain that needs it. The study and development of a new Atlas Silvaco device are taking into account the impact of several geometric and electric parameters; we focus on the electrical performances of the double gate field plate In0.2Ga0.8As/Al0.3Ga0.7As HEMT including double hetero-structure; we compare the numerical simulation using 2D Atlas Silvaco simulator with the extracted experimental results. Then we validate our model by GPR approach. The GPR approach opens promising opportunities for devices modeling without knowing too much the device physics properties. The obtained results give better performances which lead to fabricate devices with better electrical properties for promoting further investigation.
Keywords:
2D Atlas Silvaco, double hetero structure, GPR, HEMT,
References
- Abdullah, W.F.H., M. Othman, M.A.M. Ali and M.S. Islam, 2010. Improving ion-sensitive field-effect transistor selectivity with backpropagation neural network. WSEAS T. Circuit. Syst., 9(11): 700-712.
Direct Link
-
ATLAS User's Manual, 2012. Device simulation software, 2012. Silvaco International, Santa Clara, CA.
-
Benacer, I. and Z. Dibi, 2014. Modeling and simulation of organic field effect transistor (OFET) using artificial neural networks. Int. J. Adv. Sci. Technol., 66: 79-88.
CrossRef
-
Bhatia, V., N. Pandey and A. Bhattacharyya, 2016. Modelling and design of inverter threshold quantization based current comparator using artificial neural networks. Int. J. Elect. Comput. Eng., 6(1): 320-329.
CrossRef
- Chan, L.L.T., Y. Liu and J. Chen, 2013. Nonlinear system identification with selective recursive Gaussian process models. Ind. Eng. Chem. Res., 52(51): 18276-18286.
CrossRef
- Chen, Y.C., R. Lai, D.L. Ingram, T. Block, M. Wojtowicz, P.H. Liu, H.C. Yen, A. Oki, D.C. Streit and K. Yano, 2000. Highly efficient high power InP HEMT amplifiers for high frequency applications. Proceeding of the 58th IEEE DRC Devices Research Conference, pp: 139-140.
CrossRef
- Hayati, M. and B. Akhlaghi, 2013. An extraction technique for small signal intrinsic parameters of HEMTs based on artificial neural networks. AEU Int. J. Electron. Commun., 67(2): 123-129.
CrossRef
-
Hayati, M., A. Shamkhani, A. Rezaei and M. Seifi, 2008. HEMT Transistor Noise modeling using generalized radial basis function. Proceeding of the IEEE International Conference on Semiconductor Electronics, pp: 509-513.
CrossRef
- Hayati, M., M. Seifi and A. Rezaei, 2010. Double gate MOSFET modeling based on adaptive neuro-fuzzy inference system for nanoscale circuit simulation. ETRI J., 32(4): 530-539.
CrossRef
-
Lai, R., X.B. Mei, W.R. Deal, W. Yoshida, Y.M. Kim, P.H. Liu, J. Lee, J. Uyeda, V. Radisic, M. Lange, T. Gaier, L. Samoska and A. Fung, 2007. Sub 50 nm InP HEMT device with fmax greater than 1 THz. Proceeding of the IEEE International Electron Devices Meeting, pp: 609-611.
CrossRef
- Lin, J.C., P.Y. Yang and W.C. Tsai, 2007. Simulation and analysis of metamorphic high electron mobility transistors. Microelectron. J., 38(2): 251-254.
CrossRef
- Liu, Y. and Z. Gao, 2015. Real-time property prediction for an industrial rubber-mixing process with probabilistic ensemble Gaussian Process Regression models. J. Appl. Polym. Sci., 132(6): 1905-1913.
CrossRef
- Murphy, E.K. and V.V. Yakovlev, 2006. RBF network optimization of complex microwave systems represented by small FDTD modeling data sets. IEEE T. Microw. Theory, 54(7): 3069-3083.
CrossRef
-
Ni, W., L. Nørgaard and M. Mørup, 2014. Non-linear calibration models for near infrared spectroscopy. Anal. Chim. Acta, 813: 1-14.
CrossRef PMid:24528654
- Rasmussen, C.E. and C.K.I. Williams, 2006. Gaussian Processes for Machine Learning. Adaptive Computation and Machine Learning. MIT Press, Cambridge, Mass, 17: 48.
Direct Link
-
Sun, A.Y., D. Wang and X. Xu, 2014. Monthly streamflow forecasting using Gaussian process regression. J. Hydrol., 511: 72-81.
CrossRef
- Wang, C., Y. Zhang, M. Guan, L. Cui, K. Ding, B. Zhang, Z. Lin, F. Huang and Y. Zeng, 2015. Specific detection of mercury(II) irons using AlGaAs/InGaAs high electron mobility transistors. J. Cryst. Growth, 425: 381-384.
CrossRef
- Wang, K., T. Chen and R. Lau, 2011. Bagging for robust non-linear multivariate calibration of spectroscopy. Chemometr. Intell. Lab., 105(1): 1-6.
CrossRef
- Yuan, J., K.S. Wang, T. Yu and M.L. Fang, 2008. Reliable multi-objective optimization of high-speed WEDM process based on Gaussian process regression. Int. J. Mach. Tool. Manu., 48(1): 47-60.
CrossRef
-
Zhao, Z., Y. Qu, Y. Zhang and X. Shen, 2013. Overview of microwave device modeling techniques based on machine learning. Int. J. Adv. Comput. Technol., 5(9): 299-306.
CrossRef
Competing interests
The authors have no competing interests.
Open Access Policy
This article is distributed under the terms of the Creative Commons Attribution 4.0 International License (http://creativecommons.org/licenses/by/4.0/), which permits unrestricted use, distribution, and reproduction in any medium, provided you give appropriate credit to the original author(s) and the source, provide a link to the Creative Commons license, and indicate if changes were made.
Copyright
The authors have no competing interests.
|
|
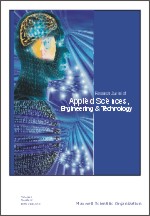 |
ISSN (Online): 2040-7467
ISSN (Print): 2040-7459 |
 |
Information |
|
|
|
Sales & Services |
|
|
|