Research Article | OPEN ACCESS
Identify and Classify Vibration Signal for Steam Turbine Based on Neural Sleep Fuzzy System
1, 2Moneer Ali Lilo, 1L.A. Latiff, 3Yousif I. Al Mashhadany and 4Aminudin Bin Haji Abu
1Razak School of Engineering and Advanced Technology, Universiti Teknologi Malaysia,
Kuala Lumpur, Malaysia
2Department of Physics, College of Science, Al Muthana University, Al Muthana
3Department of Electrical Engineering, Engineering College, University of Anbar, Ramadi, Iraq
4Malaysia-Japan International Institute of Technology, Universiti Teknologi Malaysia,
Kuala Lumpur, Malaysia
Research Journal of Applied Sciences, Engineering and Technolog 2016 5:589-598
Received: September 28, 2015 | Accepted: October 30, 2015 | Published: March 05, 2016
Abstract
Vibration in a steam turbine-generator is one of the many default problems, similar to thrust, crack and low or high speeds, all of which causes damage to the steam turbine if leaves unprotected. It leads to accidents and damages, when overcome the limit of alarm or danger zones. The protection of steam turbine generators from danger leads to reduced maintenances and augmented stability of power generation. The main proposal of this study is to identify and classify vibrations in alarm and shutdown zones, it is also intended to produce a smooth signal that can be used to adjust control value, which influences the vibration value during the start-up and power generation. We compared the series and parallel-connected Neural Network (NN) that is related to time and error to identify vibration acceleration signals and flow by sleep fuzzy sugeno system, which are designed and simulated in MATLAB. The results showed that parallel-connected NN is superior to its series-connected counterpart with vibration signals, where the Neural-Sleep-Fuzzy system and the NSFS robust system produces zero voltages when it lacks vibrations, more so after receiving a linear signal to influence nonlinear signals of vibration. This study concluded that the Artificial Intelligent (AI) system with sleep fuzzy sugeno system can be implementing to classify the fault of optimal vibration signal limitation and check the suitable treatment for this fault. Also, the analysis of results can conclude that using parallel NN is faster and more accurate compared to series NN connection.
Keywords:
Neural-fuzzy, neural network, steam turbine, vibration,
References
-
Abraham, A., 2003. Intelligent systems: Architectures and perspectives. In: Abraham, A. (Ed.), Recent Advances in Intelligent Paradigms and Applications. Springer-Verlag, Berlin, Heidelberg, pp: 1-35.
CrossRef
-
Al Mashhadany, Y.I., 2012. ANFIS-inverse-controlled PUMA 560 workspace robot with spherical wrist. Proc. Eng., 41: 700-709. http://www.sciencedirect. com/science/article/pii/S187770581202632X.
Direct Link
-
Al Mashhadany, Y., 2013. Hybrid ANFIS controller for 6-DOF manipulator with 3D model. Int. J. Comput. Technol., 4(2): 631-638.
-
Boukabou, A. and N. Mansouri, 2012. T-S fuzzy control of uncertain chaotic vibration. Shock Vib., 19: 379-389. http://www.hindawi.com/journals/ sv/2012/368207/abs/.
-
Broy, A. and C. Sourkounis, 2014. Torque control methods for active damping of vibrations in drive systems of wind turbines. Proceeding of the 9th International Conference on Ecological Vehicles and Renewable Energies (EVER), pp: 1-7.
CrossRef
-
Chan, K.H., E.J. Dozal-Mejorada, X. Cheng, R. Kephart and B.E. Ydstie, 2014. Predictive control with adaptive model maintenance: Application to power plants. Comput. Chem. Eng., 70: 91-103. http://www.sciencedirect.com/science/article/pii/S0098135414000908.
CrossRef Direct Link
-
Du, H., J. Lam, K.C. Cheung, W. Li and N. Zhang, 2013. Direct voltage control of magnetorheological damper for vehicle suspensions. Smart Mater. Struct., 22(10): 13.
CrossRef
-
Elhefnawi, M. and M. Mysara, 2012. Recurrent Neural Networks and Edited by Mahmoud ElHefnawi and Janeza Trdine 9, 51000 Rijeka, Croatia, pp: 89-114.
-
Farouk, N. and L. Sheng, 2012. Design and implementation of a fuzzy logic controller for synchronous generator., Res. J. Appl. Sci. Eng. Technol., 4(20): 4126-4131.
-
Fei, X., Z. Hao and P. Daogang, 2014. Fault diagnosis in power plant based on multi-neural network. Proceeding of the IEEE International Conference on System Science and Engineering (ICSSE), pp: 180-184.
-
Gama, C.A., A.G. Evsukoff, P. Weber, N.F.F. Ebecken and S. Member, 2008. Parameter identification of recurrent fuzzy systems with fuzzy finite-state automata representation. IEEE T. Fuzzy Syst., 16(1): 213-224.
CrossRef
-
Isaza, C.V., H.O. Sarmiento, T. Kempowsky-Hamon and M.V. LeLann, 2014. Situation prediction based on fuzzy clustering for industrial complex processes. Inform. Sciences, 279(7): 785-804. http://www.sciencedirect.com/science/article/pii/S0020025514004770.
CrossRef Direct Link
-
Jia, Y. and X.J. Liu, 2014. Nonlinear multivariable supervisory predictive control for drum-type boiler-turbine system. Proceeding of the 26th Chinese Control and Decision Conference (CCDC, 2014), pp: 1058-1063.
CrossRef
-
KangaraniFarahani, M. and S. Mehralian, 2013. Comparison between artificial neural network and neuro-fuzzy for gold price prediction. Proceeding of the 13th Iranian Conference on Fuzzy Systems (IFSC, 2013), pp: 1-5. http://ieeexplore.ieee.org/ lpdocs/epic03/wrapper.htm?arnumber=6675635.
Direct Link
-
Kazemian, H.B. and S.A. Yusuf, 2014. An ANFIS approach to transmembrane protein prediction. Proceeding of the 2014 IEEE International Conference on Fuzzy Systems (FUZZ-IEEE), pp: 1360-1365.
CrossRef
-
Londhe, P.S., B.M. Patre and A.P. Tiwari, 2014. Design of single-input fuzzy logic controller for spatial control of advanced heavy water reactor. IEEE T. Nucl. Sci., 61(2): 901-911.
CrossRef
-
Marichal, G., M. Artes and J. Garcia-Prada, 2010. An intelligent system for faulty-bearing detection based on vibration spectra. J. Vib. Control, 17(6): 931-942. http://jvc.sagepub.com/content/17/6/931. abstract.
CrossRef Direct Link
-
McKee, K.K., G. Forbes, I. Mazhar, R. Entwistle and I. Howard, 2011. A review of major centrifugal pump failure modes with application to the water supply and sewerage industries. Proceeding of the ICOMS Asset Management Conference. Gold Coast, QLD, pp: 32.
PMid:21441054
-
McKee, K.K., G.L. Forbes, I. Mazhar, R. Entwistle, I. Howard and T. Mapeza, 2012. Modification of the ISO-10816 centrifugal pump vibration severity charts for use with Octave band spectral measurements. Proceeding of the 7th Australasian Congress on Applied Mechanics (ACAM, 7), pp: 276-283.
-
McKee, K.K., G.L. Forbes, I. Mazhar, R. Entwistle, M. Hodkiewicz and I. Howard, 2015. A vibration cavitation sensitivity parameter based on spectral and statistical methods. Expert Syst. Appl., 42(1): 67-78. http://www.sciencedirect.com/science/ article/pii/S0957417414004357.
Direct Link
-
Nguyen, S.D., Q.H. Nguyen and S.B. Choi, 2015. Hybrid clustering based fuzzy structure for vibration control-Part 1: A novel algorithm for building neuro-fuzzy system. Mech. Syst. Signal Pr., 50-51: 510-525. http://www.sciencedirect.com/ science/article/pii/S0888327014001277.
Direct Link
-
Nopiah, Z.M., A.K. Junoh and A.K. Ariffin, 2015. Vehicle interior noise and vibration level assessment through the data clustering and hybrid classification model. Appl. Acoust., 87: 9-22.
CrossRef
-
Panda, S. and A.K. Patro, 2013. Adaptive neuro-fuzzy controller for thermal power plant control method. 2(6): 99-102.
-
Pani, A.K. and H.K. Mohanta, 2014. Soft sensing of particle size in a grinding process: Application of support vector regression, fuzzy inference and adaptive neuro fuzzy inference techniques for online monitoring of cement fineness. Powder Technol., 264, 484-497. http://www.sciencedirect. com/science/article/pii/S003259101400518X.
CrossRef Direct Link
-
Rong, H.J., 2012. Indirect adaptive fuzzy-neural control of robot manipulator. Proceeding of IEEE 14th International Conference on High Performance Computing and Communication and 2012 IEEE 9th International Conference on Embedded Software and Systems, pp: 1776-1781.
CrossRef' target='_blank'>CrossRef
-
Rosa, R., F. Gomide and R. Ballini, 2013. Evolving hybrid neural fuzzy network for system modeling and time series forecasting. Proceeding of the 12th International Conference on Machine Learning and Applications, pp: 378-383.
CrossRef
-
Su, H., K.T. Chong and R. Ravi Kumar, 2011. Vibration signal analysis for electrical fault detection of induction machine using neural networks. Neural Comput. Appl., 20(2): 183-194.
CrossRef
-
Wentao, H., Y. Jun, Z. Xuezeng and L. Xiaojun, 2014. Fault diagnosis for steam turbine based on flow graphs and naïve bayesian classifier. Proceeding of the IEEE International Conference on Mechatronics and Automation (ICMA, 2014), pp: 396-401.
PMCid:PMC4616388
-
Wulandhari, L.A., A. Wibowo and M.I. Desa, 2014. Condition diagnosis of multiple bearings using adaptive operator probabilities in genetic algorithms and back propagation neural networks. Neural Comput. Appl., 26: 57-65.
CrossRef
-
Xiao, H., J. Zhou, J. Xiao, W. Fu, X. Xia and W. Zhang, 2013. Identification of vibration-speed curve for hydroelectric generator unit using statistical fuzzy vector chain code and support vector machine. P. I. Mech. Eng. O-J. Risk Reliab., 228(3): 291-300. http://pio.sagepub.com/content/ early/2013/12/26/1748006X13518032.
Direct Link
-
Xie, T., H. Yu and B. Wilamowski, 2010. Replacing fuzzy systems with neural networks. Proceeding of the 3rd International Conference on Human System Interaction, 2(1): 189-193.
CrossRef
-
Yanbing, Z., L. Yibing, A. Hongwen and Y. Keguo, 2011. Fault recognition of large steam turbine based on higher order spectral features of vibration signals. Proceeding of the IEEE International Conference on Mechatronics and Automation (ICMA, 2011), pp: 1572-1577.
CrossRef
-
Yin, J., J. Wu, X. Yuan, X. Wang and Y. Fan, 2013. Study and design of diaphragm pump vibration detection fault diagnosis system based on FFT. Res. J. Appl. Sci. Eng. Technol., 5(4): 1238-1244.
-
Yue, W., Y. Cai, Q. Rong, C. Li and L. Ren, 2014. A hybrid life-cycle and fuzzy-set-pair analyses approach for comprehensively evaluating impacts of industrial wastewater under uncertainty. J. Clean. Prod., 80: 57-68. http://www.sciencedirect. com/science/article/pii/S095965261400585X.
CrossRef Direct Link
-
Zhang, Y., S. Huang, W. Gao and T. Shen, 2008. Vibration fault diagnosis of steam turbine shafting based on probability neural networks. Proceeding of Congress on Image and Signal Processing (CISP’08), pp: 582-585.
CrossRef
-
Zurada, J.M., 1992. Introduction to Artificial Neural System. West Publishing Co., St. Paul, United States of America.
Competing interests
The authors have no competing interests.
Open Access Policy
This article is distributed under the terms of the Creative Commons Attribution 4.0 International License (http://creativecommons.org/licenses/by/4.0/), which permits unrestricted use, distribution, and reproduction in any medium, provided you give appropriate credit to the original author(s) and the source, provide a link to the Creative Commons license, and indicate if changes were made.
Copyright
The authors have no competing interests.
|
|
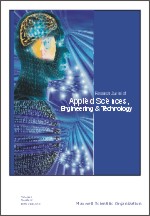 |
ISSN (Online): 2040-7467
ISSN (Print): 2040-7459 |
 |
Information |
|
|
|
Sales & Services |
|
|
|