Research Article | OPEN ACCESS
Combination of Nonlinear Feature Extraction Techniques for ECG Arryhtmia Detection System
1, 2Fatin Ahmed Elhaj, 1, 3Arief R. Harris, 3Tan Tian Swee and 2Naomie Salim
1Neural Engineering Lab, Centre for Biomedical Engineering
2Soft Computing Research Group, Faculty of Computing, Universiti Teknologi Malaysia,
Skudai 81310, Malaysia
3Faculty of Biosciences and Medical Engineering, Universiti Teknologi Malaysia, Johor, Malaysia
Research Journal of Applied Sciences, Engineering and Technology 2015 9:1024-1029
Received: June 24, 2015 | Accepted: August 2, 2015 | Published: November 25, 2015
Abstract
Electrocardiogram (ECG) is a noninvasive method used to detect arrhythmias or heart abnormalities. It describes the electrical activity of the heart. Physicians are faced with difficulties in detecting irregular heartbeats due to the presence of noise and subtle changes in the signal amplitude and duration. Depending on human visual detection alone may lead to misdiagnosis or insignificant detection of cardiovascular diseases. A computer-aided diagnosis of the ECG will assist physicians to significantly detect the cardiovascular diseases. Non-linear method is useful to extract and capture hidden information in the ECG signal. In this study we present a combination of two nonlinear methods; Higher Order Statistics (HOS) cumulants and Independent Component Analysis (ICA), performed on the dynamics ECG signals for arrhythmia detection. The abnormal heartbeats focused in this study are Right Bundle Branch Block (RBBB), Left Bundle Branch Block (LBBB), Atrial Premature Contraction (APC), Ventricular Premature Contraction (VPC) and Paced beat (P). The HOS and ICA features were fed to the Support Vector Machine (SVM) with kernel functions for automatic classification and neural network. In our study, we obtained the highest accuracy of 96.34% with Radial Basis Function (RBF) kernel and neural network.
Keywords:
ECG, feature extraction, HOS, ICA, supervised learning algorithm,
Competing interests
The authors have no competing interests.
Open Access Policy
This article is distributed under the terms of the Creative Commons Attribution 4.0 International License (http://creativecommons.org/licenses/by/4.0/), which permits unrestricted use, distribution, and reproduction in any medium, provided you give appropriate credit to the original author(s) and the source, provide a link to the Creative Commons license, and indicate if changes were made.
Copyright
The authors have no competing interests.
|
|
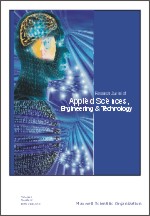 |
ISSN (Online): 2040-7467
ISSN (Print): 2040-7459 |
 |
Information |
|
|
|
Sales & Services |
|
|
|