Research Article | OPEN ACCESS
Hybrid Swarm Optimization for Cash Management Using Evolutionary Computing
1, 2A. Alli and 1M.M. Ramya
1Department of Computer Applications, Hindustan University, Chennai, Tamilnadu, India
2Department of Computer Applications, Presidency College, Bangalore, Karnataka, India
Research Journal of Applied Sciences, Engineering and Technology 10 2015 6:653-660
Received: May 16, 2015 | Accepted: June 22, 2015 | Published: October 25, 2015
Abstract
Cash forecasting plays a vital role in any financial organization to maintain the optimal cash balance to satisfy the customer needs on a daily basis without any delay. In the traditional approach statistical methods were used for cash forecasting. Banks have great challenges to avoid the surplus cash as well as to keep adequate cash to meet the customer demand. An intelligent model is needed to identify the cash requirement using cognitive approach. Hence, an evolutionary computing using the hybrid swarm system was introduced for the cash management of a bank. In this study, cash prediction models were developed from the historic short term data and long term data. In order to find the daily cash requirement of financial organization an intelligent hybrid model composed of an Artificial Neural Network (ANN) and a Particle Swarm Optimization (PSO) was introduced. The proposed methodology was capable of training and adjusting the ANN parameters through PSO to improve the efficiency of the cash management model. In a PSO-based ANN model, PSO searches for a set of best weights and biases for an ANN to minimize the error were evaluated using Mean Square Error (MSE). The experimental analysis was made for the selected parameters to maintain the optimal cash. The proposed ANNPSO model has proven its accuracy with the best MSE of short term data was 0.0035 and for long term data was found at 0.0029.
Keywords:
ANN, inertia, MSE, neurons, position, PSO, velocity,
Competing interests
The authors have no competing interests.
Open Access Policy
This article is distributed under the terms of the Creative Commons Attribution 4.0 International License (http://creativecommons.org/licenses/by/4.0/), which permits unrestricted use, distribution, and reproduction in any medium, provided you give appropriate credit to the original author(s) and the source, provide a link to the Creative Commons license, and indicate if changes were made.
Copyright
The authors have no competing interests.
|
|
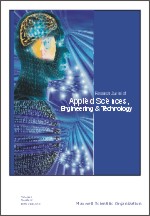 |
ISSN (Online): 2040-7467
ISSN (Print): 2040-7459 |
 |
Information |
|
|
|
Sales & Services |
|
|
|