Research Article | OPEN ACCESS
A Framework for Heart Disease Prediction Using K nearest Neighbor Algorithm
R. Kavitha and E. Kannan
Department of CSE, VEL Tech University, Chennai-62, Tamil Nadu, India
A Framework for Heart Disease Prediction Using K nearest Neighbor Algorithm 2015 1:10-13
Received: October ‎25, ‎2014 | Accepted: February ‎27, ‎2015 | Published: September 05, 2015
Abstract
Heart disease prediction is an area where many researchers are working using different data mining techniques. This study proposes a framework to develop a heart disease prediction process using k-nearest neighbor with wrapper filter. Heart disease diagnosis is mostly done with doctor�s knowledge and practice. But the cost spent by the patients are more in order to take test in which all the test does not contribute towards effective diagnosis of disease. The patient�s record is predicted to find if they have symptoms of heart disease through data mining techniques. Many researches have been undergone and researchers have been using several data mining techniques to help health care professionals in the diagnosis of heart disease. In heart disease database there exists several features out of which only few are critical features. The feature which contributes towards effective diagnosis is termed as critical feature. Our study proposes a framework to find the subset of critical feature using K nearest neighbor and wrapper filter. This in turn produces a prediction model. Finally we exhibit the ideas of diagnosing heart disease with critical feature.
Keywords:
Classification, feature, heart disease, nearest neighbor, prediction,
References
-
David, S. and T. Evangelos, 2013. On identifying critical nuggets of information during classification tasks. IEEE T. Knowl. Data En., 25(6): 1354-1367.
CrossRef - Jabbar, J.K., C. Priti and B.L. Deekshatulu, 2012. Knowledge discover from mining association rules for heart disease prediction. J. Theor. Appl. Inf. Technol., 41(2).
-
Jabbar, M.A., B.L. Deekshatulu and P. Chandra, 2013a. Heart disease classification using nearest neighbor classifier with feature subset selection. Anale Seria Inform., 9: 47-54.
- Jabbar, M.A., B.L. Deekshatulu and P. Chandra, 2013b. Classification of heart disease using artificial neural network and feature subset selection. Glob. J. Comput. Sci. Tech. Neural Artif. Intell., Version 1.0., 13(3).
-
Jyoti, S., A. Ujma, S. Dipesh and S. Sunita, 2011. Predictive data mining for medical diagnosis: An overview of heart disease prediction. Int. J. Comput. Appl., 17(8): 975-8887.
-
Kavitha, R. and E. Kannan, 2014. A methodology for heart disease diagnosis using data mining technique. Res. J. Appl. Sci. Eng. Technol., 8(11): 1350-1354.
- Koteeswaran, S., J. Janet, E. Kannan and P. Visu, 2012. Terrorist: Intrusion monitoring system using outlier analysis based search knight algorithm. Eur. J. Sci. Res., 74(3): 440-449.
- Mai, S., T. Tim and S. Rob, 2012. Applying K-nearest neighbour in diagnosing heart disease patients. Int. J. Inf. Educ. Technol., 2(3): 220-223.
- Nidhi, B. and J. Kiran, 2012. An analysis of heart disease prediction using different data mining techniques. Int. J. Eng. Res. Technol., 1(8): 1-4.
-
Rajeswari, K., V. Vaithiyanathan and P. Amirtharaj, 2011. Prediction of risk score for heart disease in India using machine intelligence. Proceeding of the International Conference on Information And Network Technology IACSIT Press, Singapore, 4: 18-22.
- Sidahmed, M., A. Baghdad and M. Mostéfa, 2013. Supervised feature selection for diagnosis of coronary artery disease based on genetic algorithm. In: Sundarapandian et al. (Eds.), CSE, CICS, DBDM, AIFL, SCOM, 1: 41-51.
Competing interests
The authors have no competing interests.
Open Access Policy
This article is distributed under the terms of the Creative Commons Attribution 4.0 International License (http://creativecommons.org/licenses/by/4.0/), which permits unrestricted use, distribution, and reproduction in any medium, provided you give appropriate credit to the original author(s) and the source, provide a link to the Creative Commons license, and indicate if changes were made.
Copyright
The authors have no competing interests.
|
|
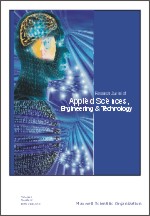 |
ISSN (Online): 2040-7467
ISSN (Print): 2040-7459 |
 |
Information |
|
|
|
Sales & Services |
|
|
|