Abstract
|
Article Information:
Content Based Mammogram Retrieval based on Breast Tissue Characterization using Statistical Features
K. Vaidehi and T.S. Subashini
Corresponding Author: K. Vaidehi
Submitted: June 17, 2014
Accepted: July 13, 2014
Published: August 20, 2014 |
Abstract:
|
The aim of the study is to retrieve the similar mammographic images based on the type of breast tissue density of the given query image. Statistical descriptors were extracted from the candidate blocks of the breast parenchyma. The mean of extracted features are fed into the SVM classifier for classification of the tissue density into any of the three classes namely dense, glandular and fatty and the classification accuracy obtained is 91.54%. After classification the mammogram images along with its feature vector are stored into three separate databases based on tissue type. Then K-means clustering algorithm is used to divide each database into 2 clusters. For content based retrieval of the mammograms based on the given query image, first the query image is classified into any of the three tissue class. Then the feature vector of the query image is compared with the two cluster centroids of the corresponding class, so as to confine the search within the closest cluster. Top 5 similar images are retrieved from its corresponding class database. Euclidean distance based k-NN is used for mammogram retrieval and this study obtained the highest precision rate ranging between 98 and 99%.
Key words: Breast tissue density, CBIR, mammography, statistical features, SVM, ,
|
Abstract
|
PDF
|
HTML |
|
Cite this Reference:
K. Vaidehi and T.S. Subashini, . Content Based Mammogram Retrieval based on Breast Tissue Characterization using Statistical Features. Research Journal of Applied Sciences, Engineering and Technology, (7): 871-878.
|
|
|
|
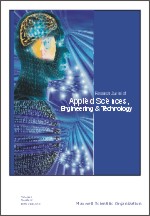 |
ISSN (Online): 2040-7467
ISSN (Print): 2040-7459 |
 |
Information |
|
|
|
Sales & Services |
|
|
|