Abstract
|
Article Information:
A Novel Optimized Adaptive Learning Approach of RBF on Biomedical Data Sets
Anusuya S. Venkatesan and Latha Parthiban
Corresponding Author: Anusuya S. Venkatesan
Submitted: April 01, 2014
Accepted: May 19, 2014
Published: July 25, 2014 |
Abstract:
|
In this study, we propose a novel learning approach of Radial Basis Function Neural Network (RBFNN) based on Fuzzy C-Means (FCM) and Quantum Particle Swarm Optimization (QPSO) to group similar data. The performance of RBFNN relies on the parameters such as number of hidden nodes, centres and width of Gaussian function and weight matrix between hidden layer and output layer. Generally, RBF is trained with a fixed number of nodes but in this study we allow the network to have variable number of hidden nodes based on the size of input samples. The clustering algorithm, Fuzzy C Means (FCM) is optimized with QPSO to provide global optimal centres for RBFNN. The weights are calculated by using Least Square Method and the root mean square error is optimized to improve the accuracy, accordingly the hidden unit numbers are adjusted. The cluster centres are obtained using optimized FCM and are checked against random selection of centres to verify the suitability. The datasets such as liver disorder and breast cancer from UCI machine learning repository are used for the experiments. The accuracy is analyzed for the Cluster Numbers (CN) 2, 3, 4, 5, 6, 7, 8, 9, 10, 15 and 20, respectively.
Key words: FCM, fitness, QPSO, RBFNN, RMSE , ,
|
Abstract
|
PDF
|
HTML |
|
Cite this Reference:
Anusuya S. Venkatesan and Latha Parthiban, . A Novel Optimized Adaptive Learning Approach of RBF on Biomedical Data Sets. Research Journal of Applied Sciences, Engineering and Technology, (4): 541-547.
|
|
|
|
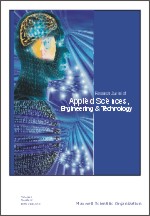 |
ISSN (Online): 2040-7467
ISSN (Print): 2040-7459 |
 |
Information |
|
|
|
Sales & Services |
|
|
|